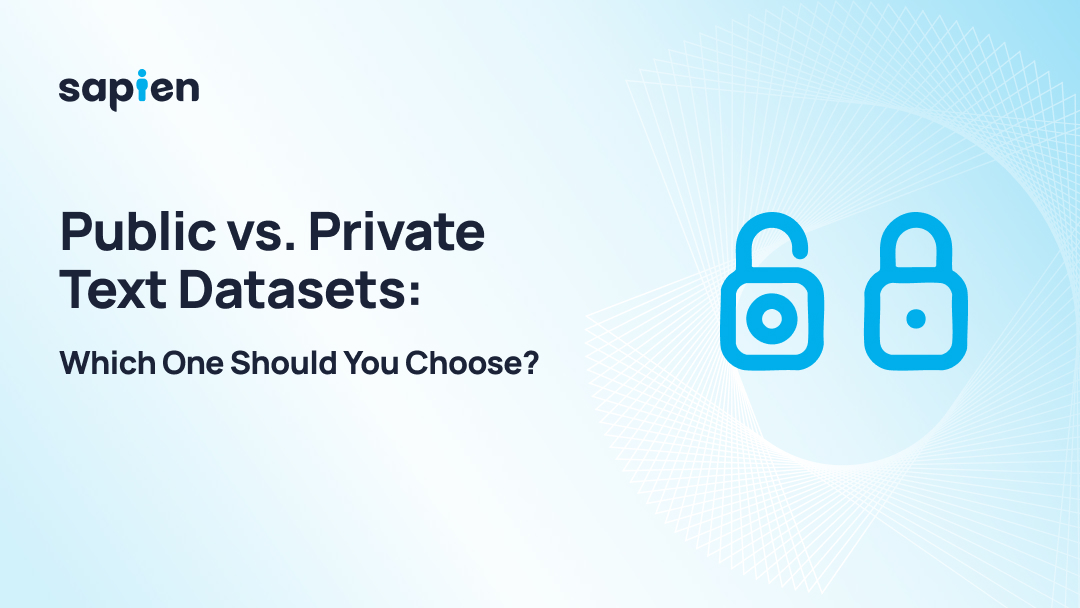
为了构建准确、有效的模型,人工智能系统在很大程度上依赖于训练它们所使用的数据集。在考虑数据集时,人工智能专业人员经常面临一个关键的选择:他们应该使用公共文本数据集还是私有文本数据集?这一决定可能会对研究成果、产品开发和模型性能产生重大影响。
本文将帮助您了解公共和私有文本数据集之间的区别、它们各自的优势和局限性,以及如何根据您的特定需求选择正确的文本数据集。
关键要点
- 公共数据集:免费提供的数据集非常适合一般研究、训练 AI 模型和测试算法。非常适合大规模任务和学术研究,但可能需要大量的预处理。
- 私有数据集:针对特定业务需求量身定制的专有数据集,提供高质量、安全和机密的数据。最适合特殊任务,但成本高昂且难以访问。
- 主要区别:公共数据集易于访问且具有成本效益,但可能缺乏特异性,需要更多的预处理。私有数据集高质量且可自定义,但成本更高且有访问限制。
- 混合方法:将公共和私有数据集结合可以最大限度地发挥两者的优势,平衡成本、可扩展性和量身定制的见解,以提高模型性能。
什么是公共文本数据集?
公开 文本数据集 是免费向公众提供的文本数据的集合。这些数据集可用于各种目的,例如研究、训练 AI 模型或测试算法。
公共数据集的优势
公共数据集具有多种优点,使其对各种应用程序具有吸引力。以下是主要优势:
- 可访问性:公共数据集可免费使用,任何人都可以访问,从而促进了开放协作。
- 透明度:它们使研究人员能够复制和验证结果,从而更容易验证发现并为科学进步做出贡献。
- 大规模数据:许多公共数据集非常广泛,包含大量数据,非常适合训练深度学习模型。
- 社区支持:公共数据集的开源性质鼓励研究人员之间的协作和见解共享,这可以推动创新。
公共数据集的局限性
尽管有许多优点,但使用公共数据集会带来一些挑战。以下是主要限制:
- 预处理要求:公共数据集通常需要大量的清理和预处理,以使其可用于特定任务。
- 质量和相关性:一些公共数据集可能包含噪音、无关紧要或过时的数据,这可能会降低基于这些数据集构建的模型的有效性。
- 潜在的偏见:公共数据集可以反映其来源的固有偏差,这可能会影响机器学习模型的准确性和公平性。
数据收集中的偏见 是公共数据集偏差的最常见贡献者之一。由于这些数据集通常是从开放论坛、社交媒体或众包平台汇总而成的,因此它们可能会无意中夸大了某些群体,而低估了其他群体,从而导致模型在不同人群中表现不佳。
什么是私有文本数据集?
私有文本数据集是个人、组织或公司拥有的专有数据集。与公共数据集不同,私有数据集通常是为了满足特定的业务需求或研究要求而精心策划的。
私有数据集的优势
私有数据集具有多种明显的优势,特别是对于寻求量身定制的解决方案或独特见解的企业而言。以下是主要好处:
- 定制:私有数据集可以根据特定的业务需求量身定制,提供公共数据集所没有的独特见解。
- 质量:这些数据集通常针对特定任务进行清理、整理和优化,从而生成可供分析或模型训练的高质量数据。
- 竞争优势:由于私有数据集不公开,公司可以通过使用其他人无法访问的数据来获得竞争优势。
- 隐私和安全:使用私有数据集,可以更安全地处理敏感信息,从而确保专有数据和客户信息的机密性。
私有数据集的局限性
尽管私有数据集有很多好处,但也有一些挑战需要考虑。以下是主要限制:
- 成本:获取和维护私有数据集可能很昂贵,尤其是在需要持续更新的情况下。
- 可访问性:访问私有数据集通常需要特殊权限或保密协议 (NDA),这可能会成为某些项目的障碍。
- 缩小规模:私有数据集可能不如公共数据集大,这可能会限制其对需要大量数据的大规模 AI 模型的用处。
在公共数据集和私有数据集之间进行选择的关键注意事项
在公共文本数据集与私有文本数据集之间做出决定时,评估项目的需求至关重要。实际上,最近的 Gartner 的研究 表明了这一点 75% 的人工智能项目失败是由于数据质量差或数据集不合适造成的,这凸显了从一开始就选择正确的数据类型的重要性。以下是需要考虑的关键因素:
在公共和私有文本数据集之间进行选择最终取决于您的数据与项目范围、隐私要求和特定目标的匹配程度。这不仅仅是可用性或规模的问题,还关系到战略相关性。正如谷歌首席决策科学家卡西·科济尔科夫所说:
“更好的数据每次都胜过更多的数据。这不是要为你的模型提供大量信息,而是要为他们提供正确的信息。”
这种观点突显了一个关键要点:在选择数据集时,质量和情境匹配度应大于数量。无论你是将大型公共数据集用于广泛的研究目的,还是投资为利基应用量身定制的私有数据集,你的人工智能模型的有效性都取决于做出有意的、与目标一致的数据选择。
每种数据集类型的真实场景
了解何时使用公共文本数据集与私有文本数据集对于 AI 的成功至关重要。在使用私有或 公共数据集 通常取决于项目的具体目标,无论是学术研究、商业智能还是两者的结合。以下是一些现实场景,重点介绍了如何利用每种数据集类型。
知道何时使用公共数据集与私有数据集可以显著影响 AI 或数据驱动项目的成功。
在学术研究中使用公共数据集
学术研究人员经常依靠大型公共数据集进行研究和验证等领域的算法 自然语言处理 (NLP) 或情感分析。例如,研究人员可能会使用公开的情感分析数据集来训练一个检测文本情感基调的模型。
在商业智能中使用私有数据集
在商业领域,公司经常使用私有数据集来深入了解客户行为、偏好或反馈。例如,一家公司可能会使用客户评论的私有数据集来训练一个模型,该模型可以预测未来的购买行为或生成个性化的产品推荐。这种方法正变得越来越普遍;a 全球研究 透露,大约90%的公司认为他们可以从使用大数据中受益。
合并公有和私有数据集
结合公有和私有数据集优势的混合方法通常可以带来更全面的见解。例如,您可以使用公共数据集来收集有关某个主题的一般知识,而私有数据集则提供更具体、量身定制的见解,可以提高模型性能。
使用 Sapien 为您的项目选择合适的数据集
在公共数据集和私有数据集之间做出决定时,必须评估项目的独特需求,无论是学术研究、商业智能还是专业应用程序。公共数据集为一般任务提供可访问性和大规模数据,而私有数据集则提供量身定制的高质量见解,对业务特定需求至关重要。但是,这两种类型都有成本和局限性,需要仔细考虑。
无论您是进行学术研究、训练 AI 模型还是收集业务见解,Sapien 都可以优化数据集处理,并确保您的项目尽可能利用最佳数据。通过结合公共和私有数据集的优势,Sapien允许您采用混合方法,增强模型性能并轻松推动创新。
如果您想就数据做出更明智的决策并提高人工智能驱动项目的效率,请探索 Sapien 今天如何提升您的数据集管理策略。
常见问题解答
公共文本数据集和私有文本数据集的主要区别是什么?
公共数据集是开放访问和免费提供的,而私有数据集是专有的,通常是为特定任务精心策划的,而且需要付出一定的代价。
我可以合并公共和私有数据集来训练模型吗?
是的,结合这两种类型可以利用公共可访问性和私人定制的优势,提供更加平衡和全面的数据集。
公共数据集总是可以免费使用吗?
是的,公共数据集通常是免费的,但在使用前可能需要额外的预处理和验证。