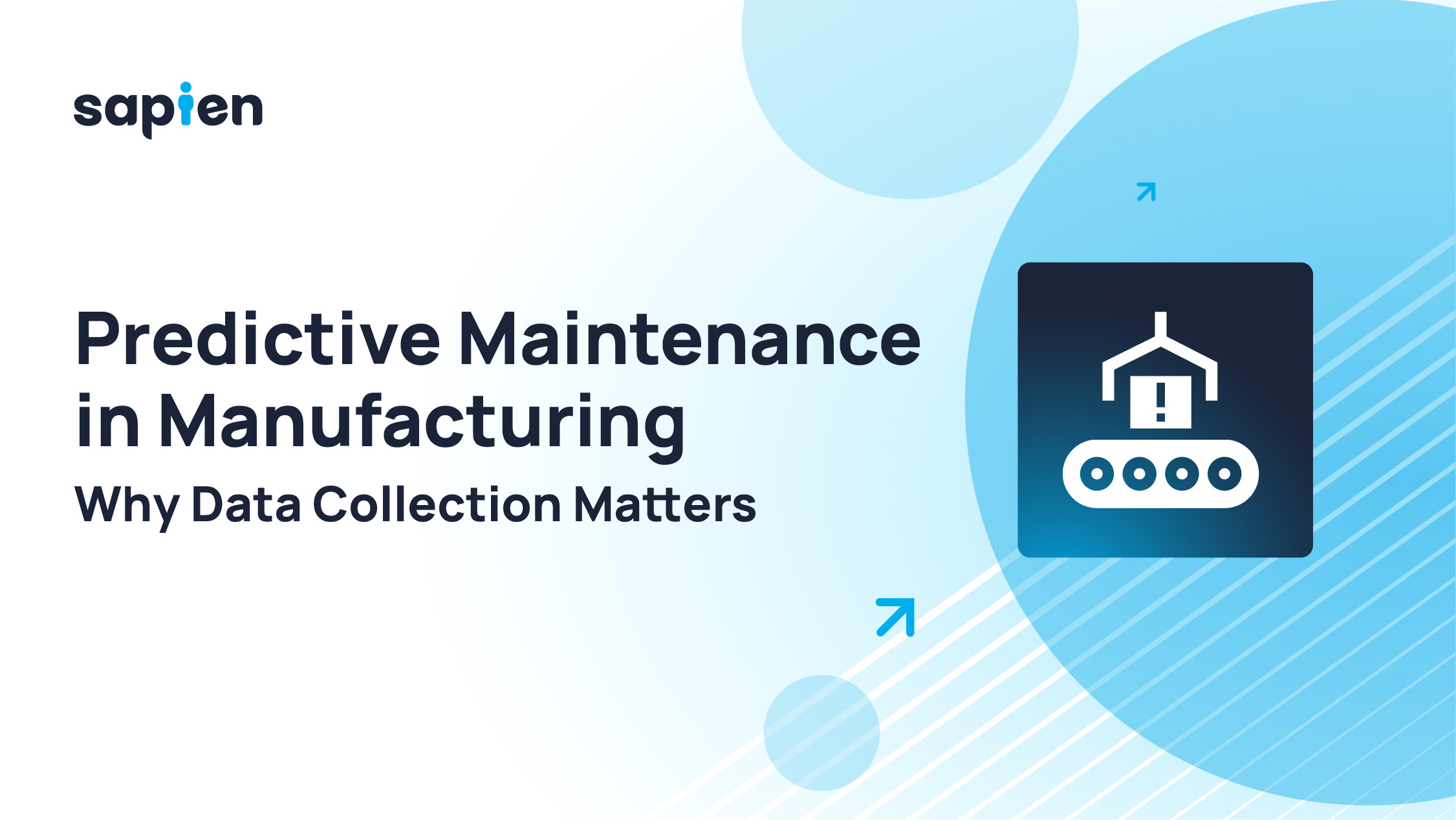
In the modern manufacturing industry, one of the most significant advancements in optimizing operational performance is the use of data-driven decisions. With the advent of technology like IoT, AI, and machine learning, predictive maintenance has emerged as a game-changer in ensuring efficiency, reducing downtime, and lowering operational costs.
This article explores the role of data collection in predictive maintenance, how it differs from traditional maintenance methods, and how manufacturers can implement it to improve their operations.
Key Takeaways
- Data-driven decisions: Data enables manufacturers to optimize operations, reduce inefficiencies, and enhance resource allocation across the production line.
- Predictive maintenance: Unlike reactive or preventive maintenance, predictive maintenance uses real-time data to anticipate failures and minimize downtime.
- Sensor integration: IoT sensors monitor machine conditions continuously, capturing metrics like temperature and vibration to detect anomalies early.
- AI and machine learning: Algorithms analyze large datasets to identify patterns, enabling accurate failure predictions and optimized maintenance schedules.
- Implementation challenges: Issues like data quality, system integration, and cybersecurity must be addressed for predictive maintenance to succeed.
- Competitive edge: Companies that invest in predictive technologies improve asset management and gain a long-term advantage in the manufacturing sector.
Importance of Data-Driven Decisions in Modern Manufacturing
Data is at the heart of industrial innovation today. In manufacturing, data-driven decisions help companies understand their systems more deeply, leading to optimized processes and resources. With data, manufacturers can:
- Identify inefficiencies before they cause significant downtime.
- Predict potential failures in machinery, enabling timely repairs and replacements.
- Optimize production lines, ensuring consistent output with minimal waste.
As manufacturing operations become more complex and interconnected, the need for precise data collection and analysis grows exponentially.
Predictive Maintenance with MATLAB: A Data-Based Approach
The Role of Data Collection in Predictive Maintenance
Predictive maintenance uses data to foresee failures before they occur. Unlike traditional maintenance, which often relies on scheduled or reactive repairs, predictive maintenance uses real-time data collected from various sensors embedded in machinery and equipment. This data helps monitor the condition of machines continuously, enabling manufacturers to predict when and why equipment will fail.
In this system, data collected is analyzed through advanced algorithms to identify patterns and anomalies that might indicate impending failures. By anticipating when maintenance is required, manufacturers can avoid unplanned downtime and costly repairs. A McKinsey report also found that predictive maintenance can reduce machine downtime by up to 50% and extend the life of aging equipment by 20–40%.
Traditional Maintenance vs. Predictive Maintenance
Traditional maintenance methods can be categorized into two types: reactive maintenance and preventive maintenance.
- Reactive Maintenance: This approach deals with repairing machines only after they break down, leading to costly downtime and urgent repair costs.
- Preventive Maintenance: Maintenance is performed at regular intervals, regardless of the condition of the equipment, which may lead to unnecessary maintenance or missed opportunities for more efficient repairs.
In contrast, predictive maintenance improves operational efficiency by using real-time data to predict equipment failures, allowing manufacturers to take action only when needed. This results in reduced maintenance costs and better asset management.
Benefits of Predictive Maintenance
The benefits of predictive maintenance are vast, especially when it comes to reducing costs and improving overall efficiency:
- Reduced Downtime: By predicting when a machine will fail, companies can schedule maintenance without disrupting the production process.
- Lower Repair Costs: Timely repairs prevent extensive damage to equipment, lowering repair costs.
- Extended Equipment Life: Regular, data-driven maintenance ensures machines are operating optimally, extending their lifespan.
- Increased Productivity: With fewer unplanned downtimes, production runs smoother and more efficiently.
Importance of Data in Predictive Maintenance
Data is the backbone of predictive maintenance. Various types of data are collected from different parts of the manufacturing system to build predictive models. Some of the most critical types of data include:
- Operational Data: This includes real-time metrics like production speed, energy consumption, and machine performance.
- Environmental Data: Environmental factors, such as temperature, humidity, and pressure, can impact machinery performance and are integral to the predictive model.
- Machine-Generated Data: Data generated directly by machines, such as vibrations, motor conditions, and wear patterns, helps identify potential failures.
With this data, machine learning models can forecast failures based on historical trends, optimizing both maintenance schedules and production processes.
Key Data Collection Methods for Predictive Maintenance
Effective data collection for predictive maintenance involves several methods, each designed to capture critical data from the machinery.
Sensors and IoT Devices
The Internet of Things (IoT) has revolutionized manufacturing by enabling real-time monitoring of equipment. IoT sensors embedded in machines capture data like temperature, vibration, and pressure, sending this information back to a central system. These sensors are crucial for understanding the health of equipment.
Machine Learning and AI Algorithms
Machine learning and AI algorithms analyze the data collected from sensors to predict equipment failure. By processing vast amounts of data, AI can identify patterns not immediately apparent to human operators.
AI models are used to forecast failures in pumps, motors, and other critical components, ensuring timely interventions before major breakdowns occur.
Manual Data Collection
While IoT devices and AI are powerful tools, manual data collection still plays a role in predictive maintenance. Operators can provide valuable insights through:
- Operator Reports: Human inspection can detect signs of failure not caught by sensors.
- Manual Inspections: Regular checks and maintenance logs help identify issues that may not be immediately apparent from automated data.
Integrating both automated and manual data enhances predictive accuracy.
Challenges in Data Collection for Predictive Maintenance
Though predictive maintenance offers many benefits, it is not without challenges. Manufacturers must consider several factors when implementing data collection strategies:
- Data Quality and Accuracy: For predictive models to be effective, the data used must be high quality. This includes ensuring sensors are calibrated and data is validated regularly to avoid false predictions.
- Integration of Data from Multiple Sources: With numerous machines and sensors, data is often scattered across multiple platforms. Integrating this data into a single, cohesive system is crucial for effective decision-making.
- Data Privacy and Security: As manufacturers collect vast amounts of operational data, ensuring the privacy and security of this data is essential. Compliance with regulations, such as GDPR, is critical.
Transform Maintenance with High-Quality Data from Sapien
Data is revolutionizing manufacturing - especially in predictive maintenance - by enabling manufacturers to anticipate failures, minimize downtime, and maximize operational efficiency through intelligent analysis of machine, environmental, and operational data.
To stay competitive in a rapidly evolving industry, forward-thinking manufacturers must invest in robust data collection and predictive maintenance technologies. Sapien’s data collection services empower you with high-quality, actionable datasets tailored for industrial use cases - so you can streamline operations, cut maintenance costs, and extend equipment lifespan with confidence.
Ready to future-proof your manufacturing operations? Partner with Sapien to harness the power of precision data collection and take your predictive maintenance strategy to the next level.
FAQs
What kind of sensors are used in predictive maintenance?
Sensors like temperature, vibration, pressure, and current/voltage sensors are used to monitor equipment health. These sensors collect real-time data that helps predict failures and ensure timely maintenance.
What industries besides manufacturing use predictive maintenance?
Besides manufacturing, predictive maintenance is widely used in energy, transportation, aerospace, and oil and gas, helping these industries optimize operations and reduce risks.
Can small and mid-sized manufacturers afford predictive maintenance solutions?
Yes, many predictive maintenance solutions are scalable and affordable, especially with cloud-based platforms and entry-level solutions that reduce upfront costs, making them accessible for smaller businesses.