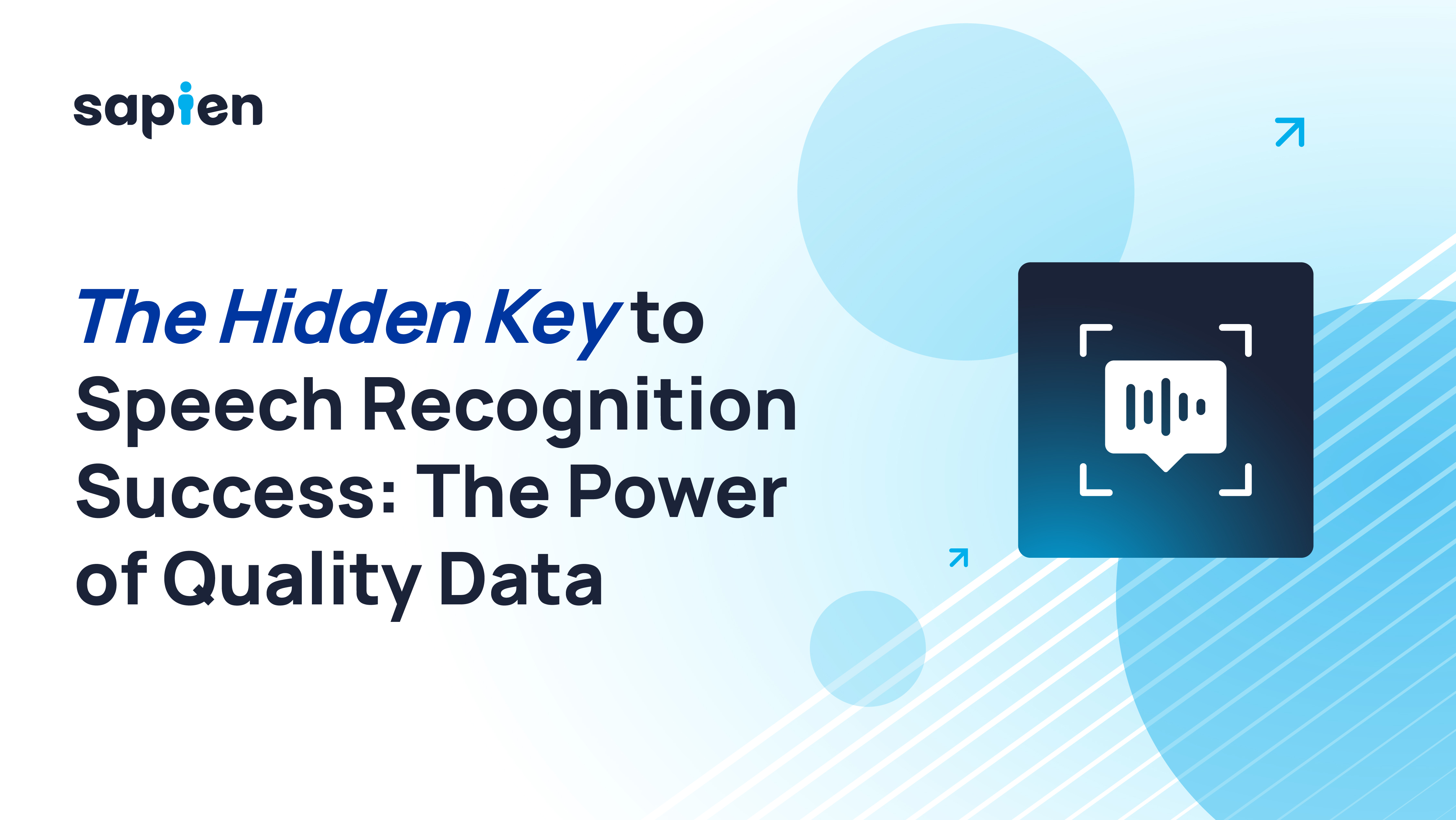
The success of speech recognition systems is directly linked to the quality of the datasets used to train them. These systems are employed in a variety of applications, from voice assistants to automated transcription services, call centers, and real-time translation tools. If you are building or improving such a system, understanding the importance of high-quality audio datasets will be crucial.
This article explores how to create dataset for speech recognition, the essential components that contribute to its effectiveness, and how emerging trends are reshaping the landscape.
Key Takeaways
- Diversity and Inclusion: Speech recognition models need diverse and inclusive datasets to accurately understand various speech patterns, accents, and dialects.
- Core Dataset Elements: High-quality audio, precise labeling, and dataset diversity are foundational to effective speech model training.
- Challenges in Dataset Creation: Issues like overfitting and annotation bottlenecks pose significant hurdles and call for creative, scalable solutions.
- Emerging Trends: The future of speech recognition lies in the rise of synthetic data, integration of multimodal datasets, and the power of crowdsource-driven models.
The Role of Datasets in Speech Recognition
Speech recognition systems rely on high-quality audio datasets to train and optimize their performance. As Dr. Xenia Karpov, an AI research scientist specializing in speech processing, states,
The diversity and quality of your dataset is what separates a model that works from one that fails.
For a model to be effective, the dataset for speech recognition must be rich in varied speech patterns, accents, dialects, and environmental conditions.
A well-constructed dataset will enable the system to:
- Understand different accents and dialects.
- Accurately transcribe speech even in noisy environments.
- Handle a variety of speech patterns and speaking speeds.
For instance, in healthcare, it is crucial that a speech recognition system can accurately transcribe medical terminology, even when spoken with a variety of accents or in noisy hospital environments.
Key Elements of a High-Quality Audio Dataset
Building audio datasets is a complex process that requires attention to several key elements. These elements are foundational to ensuring that your speech recognition model can handle a wide range of real-world speech inputs.
1. Data Diversity
A diverse dataset ensures that the model can handle different types of speech and environmental conditions. The dataset should ideally cover the following aspects:
2. Audio Quality
Audio quality is a crucial aspect of dataset creation. Low-quality recordings can lead to inaccuracies in transcription, which in turn affects the performance of speech recognition systems. According to Institute of Speech Technology (IST) speech recognition systems trained with audio samples at 16 kHz had a 22% higher accuracy rate compared to systems trained on lower-quality audio.
Here are some important audio specifications for optimal quality:
Techniques like noise reduction, echo cancellation, and high-fidelity recording equipment can improve audio clarity, reducing distortions that hinder model accuracy.
3. Accurate and Consistent Labeling
Accurate data labeling is a critical step in building a high-quality audio dataset. Inconsistent or inaccurate labeling can significantly degrade the performance of a speech recognition system. Labels should be applied consistently, including:
- Speaker Labels: Identifying who is speaking in the dataset.
- Punctuation: Including proper punctuation in transcriptions to ensure clarity.
- Metadata Tags: Recording details about the context in which the speech was recorded, such as the speaker’s demographics or the environmental conditions.
Building High-Quality Audio Datasets
Once you understand the importance of data quality, the next step is building audio datasets. The process involves choosing the right data collection strategies, ensuring scalability, and maintaining quality control throughout the process.
1. Collection Methods
There are various strategies for gathering high-quality audio data:
- Crowdsourcing: Leveraging platforms that allow contributors from around the world ensures a wide variety of speech patterns, accents, and environmental conditions. Crowdsourcing ensures that the dataset is representative of real-world speech and helps overcome the challenge of collecting diverse data.
- Open Datasets: For general-purpose speech recognition tasks, open-source dataset are often used. These provide large volumes of transcribed data but may lack the specific domain content needed for specialized applications.
- Custom Data Creation: For highly specialized applications, such as medical transcription or customer support systems, creating custom datasets tailored to specific use cases is necessary. For example, recording interactions between healthcare professionals and patients ensures that speech recognition models can accurately handle medical terminology and jargon.
2. Ethical and Legal Safeguards
When collecting data, it’s essential to follow ethical guidelines:
- Obtain Informed Consent: Ensure that all contributors understand how their data will be used and agree to participate.
- Anonymize Data: Remove any personally identifiable information from the recordings to protect contributors’ privacy.
- Ensure Demographic Diversity: Include diverse speakers in terms of gender, age, and accents to avoid bias in your dataset.
3. Bias Mitigation
Efforts must be made to ensure datasets represent diverse groups without favoring any particular demographic. Sapien addresses this challenge with a matching engine that assigns tasks to labelers based on skills, experience, and trust scores, ensuring fairness across datasets.
Challenges in Building High-Quality Audio Datasets
Creating high-quality audio datasets is not without its challenges. From data scarcity to annotation bottlenecks, several obstacles can impede the process of building effective datasets.
1. Data Scarcity
In some cases, high-quality data is simply unavailable. Languages with fewer speakers or specific dialects often have very little publicly available data. The challenge of sourcing high-quality audio for these languages requires innovative approaches, such as crowdsourcing and leveraging multilingual platforms to gather data from diverse contributors worldwide.
2. Annotation Bottlenecks
Manual transcription and annotation of audio data can be slow and labor-intensive. This bottleneck is particularly problematic for large datasets, which can take weeks or even months to label accurately. By using semi-automated systems or platforms with Human-in-the-Loop (HITL) capabilities, the process can be accelerated while maintaining accuracy.
3. Overfitting Risks
A common issue when training speech recognition models is overfitting, where the model performs well on the training data but fails to generalize to new, unseen data. This happens when the dataset lacks variety or is too homogeneous. To mitigate overfitting, it’s important to include a variety of speech types, environments, and contexts in the dataset and regularly update it with new data.
Future Trends in Audio Datasets
As speech recognition technology continues to advance, new trends are emerging in how audio datasets are created and used. These trends aim to make datasets more comprehensive, accurate, and scalable.
Synthetic Data
AI-generated voices, such as those created by text-to-speech models, are becoming more common in augmenting training datasets. Synthetic data allows for the rapid expansion of datasets, especially when real-world data is limited. However, synthetic data should be used cautiously, as it may not fully capture the nuances of human speech. Dr. Michael Li, a leader in speech AI research, notes.
"Synthetic data provides an opportunity to scale datasets exponentially, especially when real-world data is limited."
Multimodal Fusion
Future speech recognition models may combine audio with other types of data, such as visual information (e.g., lip-reading) or contextual cues (e.g., user intent). Integrating multimodal data will help systems understand speech in more complex environments and improve accuracy in noisy or unclear situations.
Crowdsource-Driven Models
A decentralized approach to data labeling, where a global community continuously contributes to and refines datasets, is gaining traction. This approach helps ensure that datasets are always up-to-date and reflect real-world speech patterns. Incentivizing contributors through rewards is a key feature of this model.
Building Better Speech Recognition Models with Sapien
As the demand for speech recognition systems grows, so will the need for high-quality audio datasets. Whether you're leveraging open datasets, crowdsourcing, or building custom data, it’s crucial to start with a strong foundation. Utilize decentralized platforms, embrace new data collection techniques, and refine your dataset regularly to stay ahead.
Sapien offers a scalable, high-quality audio data collection solution with diverse contributors, customizable workflows, and built-in quality checks. Leverage our global network to ensure your dataset is ready for the next generation of speech recognition systems.
FAQs
How much audio data is needed to train a speech recognition model?
For general use cases, several hundred hours may be enough, but for complex tasks, thousands of hours are ideal. The more diverse, the better.
Can AI-generated voices be used for training?
Yes, but they should supplement real-world data to ensure accuracy. AI-generated voices often lack the subtle variations in tone and emotion seen in human speech.
What is the most common challenge in speech recognition dataset creation?
Data scarcity for underrepresented languages and dialects is a significant challenge. Additionally, high-quality labeling remains a bottleneck.
How do I ensure my dataset is unbiased?
Ensure your dataset includes diverse speakers and environments. Use tools that assess and mitigate bias during data collection.