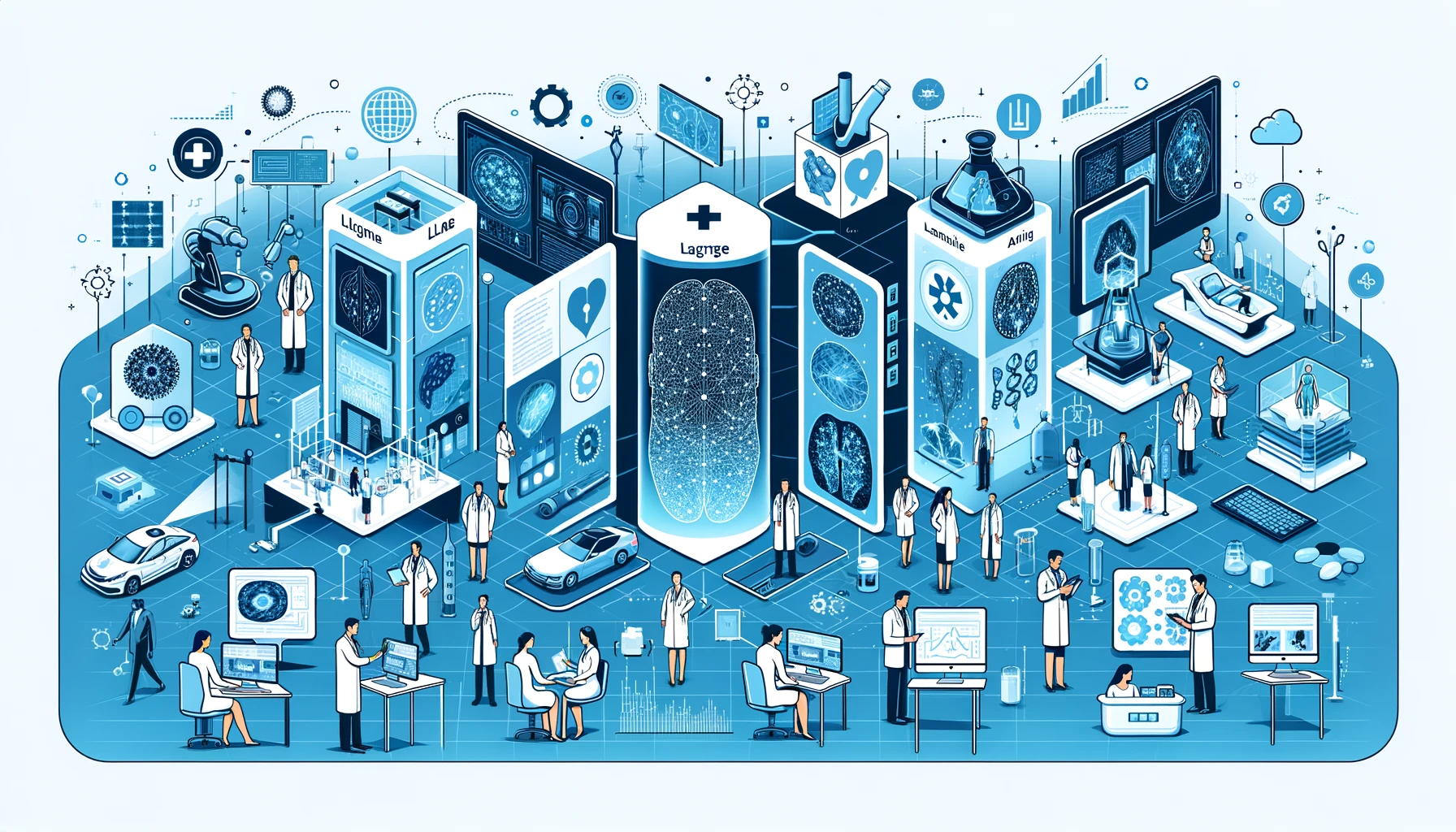
Large language models (LLMs) are now one of the leading new technologies in the field of medicine. These powerful artificial intelligence systems, trained on massive datasets of text, can interpret complex medical data, assist clinicians, further scientific research, and more. LLMs have the potential to revolutionize how we prevent, diagnose, and treat disease, and with the right data labeling services, be fine-tune for the healthcare sector in new and innovative ways.
Interpreting and Making Sense of Medical Data
One of the most promising applications of LLMs is their ability to find patterns and derive insights from huge sets of patient data. Electronic health records (EHRs) contain a wealth of information about patients' conditions, treatments, and outcomes over time. But mining value from EHRs is challenging due to their unstructured nature and sheer scale. LLMs can read hundreds of thousands of clinical notes and lab reports to reveal important trends.
For example, an LLM could analyze years of records to identify how disease symptoms progress in a population over time. This can elucidate the natural history of a condition. LLMs can also pinpoint risk factors for adverse outcomes by comparing health data between patient groups. In addition, they may discover previously unknown associations between medications, genetics, comorbidities, and disease. These kinds of insights can lead to better screening, earlier diagnosis, and more effective care. To enhance these capabilities, you can fine-tune LLM with domain-specific datasets to improve performance and accuracy for medical applications.
Accelerating Biomedical Research
The volume of published studies and clinical trials grows massively year after year. LLMs have the capacity to read millions of research papers to synthesize knowledge, generate hypotheses, and identify promising directions. This helps scientists keep up with expansive literatures and speeds the pace of discovery.
LLMs can also analyze massive datasets from genetic studies, clinical trials, and patient records to recognize patterns difficult for humans to discern. Some companies are using LLMs to identify novel drug candidates by screening libraries of compounds. The ability to extract insights from huge and varied corpuses of data makes LLMs invaluable to research.
Automating Clinical Documentation
Recording patient encounters in the medical record is time-consuming yet crucial. LLMs can capture key elements from physician notes, test results, and patient history to automatically generate clinical documentation. For instance, they can produce progress notes, discharge summaries, referral letters, and other records, freeing clinicians to focus on direct patient care.
LLMs can also ensure documentation adheres to institutional policies and billing guidelines. And the models can be continually updated to reflect new medical knowledge and each organization's preferred format and terminology. Automated clinical writing has the potential to significantly ease the documentation burden on healthcare teams.
Enhancing Medical Education
LLMs are poised to transform how we train medical professionals thanks to their ability to generate unlimited personalized educational content. Learners can get explanations of key concepts, practice making diagnoses, and test their knowledge simply by querying an LLM.
For example, medical students could describe a patient's symptoms to the LLM and ask which conditions are most likely. The model can draw on its medical knowledge to provide differential diagnoses and teach logical clinical reasoning. LLMs can also generate realistic patient cases at different difficulty levels to allow trainees to hone their skills. Interactive education, powered by a knowledge-based system, promises learning that is engaging, effective, and customized, ultimately enhancing information retention over the long term.
Improving Clinical Decision Support
Clinicians must frequently make difficult decisions about diagnosis and treatment. LLMs can serve as invaluable aids by offering data-driven recommendations at the point of care. After ingesting a patient's chart, test results, and medical history, the LLM can supply evidence-based next steps tailored to the case at hand.
It could highlight which imaging study or lab test to order that will best clarify a diagnosis based on the patient's presentation. Or it may suggest the optimal medication and dosage considering the person's genomic data, drug metabolism, comorbidities, and past response to treatments. By integrating massive knowledge and patient-specific data, LLMs can enhance clinical decision making and outcomes.
Challenges to Thoughtfully Implementing LLMs
While the potential of LLMs in medicine is impressive, effectively developing and applying them poses some challenges:
- The models rely on massive training datasets which may contain inherent biases. LLMs could perpetuate or exacerbate skewed algorithms leading to unequal outcomes for certain demographics. Addressing bias will require diverse data and constant vigilance.
- Transparency is key to build clinician and patient trust in LLM recommendations. The models must provide explanations for their outputs. Understanding how LLMs arrive at results is critical.
- Patient privacy must be paramount. Strict protocols need to govern LLM access to medical data. Only the minimum necessary information should be provided to generate useful insights while protecting confidentiality.
- Regulatory frameworks are required to ensure the safety and efficacy of LLMs if they are to be incorporated into clinical care. Extensive testing and validation are essential pre-deployment.
High-Quality Data Labeling Enables Building Robust Medical AI
A key prerequisite for developing accurate AI systems for healthcare is ensuring the data used for training is comprehensively and correctly labeled. Human labeling of medical datasets is crucial for establishing ground truth and evaluating LLM model performance. This process transforms raw data into the supervised learning needed for robust clinical AI.
Experts like board-certified physicians must carefully review and label medical images, waveforms, notes, and other data with the correct diagnoses, findings, and interpretations. For example, cardiologists need to label ECG strips with any arrhythmias present. Radiologists have to delineate anatomical structures and mark abnormalities in scans. Likewise, clinicians should annotate clinical notes to identify symptoms, medications, procedures, and family history.
This precise labeling allows AI models to learn associations between the data and labels during training. Broad clinical expertise is required to provide accurate labels and account for nuances in the data, while the tasks can be broken down for complex tasks to be completed by subject experts and the more basic tasks to be handled by non-experts. High-quality data labeling avoids incorrectly teaching the AI and enables the development of reliable, effective systems.
Strategies for Ensuring Complete, Precise Data Labels
Maintaining consistency and accuracy in data labeling at scale is challenging. Thoughtfully designed protocols and workflows are imperative.
Firstly, detailed annotation guidelines that captures all possible cases are required to standardize labels. Quality assurance protocols should also be instituted, for example having a second clinician review samples of labeled data. Inter-rater reliability metrics can then quantify annotation consistency.
Of course, the user interface for presenting data and entering labels must be intuitive and efficient for the workflow. Tools that allow directly marking up regions of interest on medical images facilitate precise localization.
On top of this, recruiting expert annotators knowledgeable in the problem is key. For example, labeling diabetic retinopathy requires optometrists trained in identifying it. Continual evaluator training and certification maintains skills. However tasks should be broken down in a grnaular way so that generalized information can be labeled by different labelers than the experts labeling the most complex parts of teh dataset.
Finally, iterative improvement of guidelines and tools based on feedback enables continuous refinement of the labeling process. As datasets grow, new corner cases will emerge requiring adjustments.
Outcomes of High-Quality Data Annotation
Investing in meticulous data labeling pays dividends in terms of the performance and utility of resulting medical AI systems. Comprehensively annotated datasets allow training more accurate, robust models.
In addition, proper documentation of the annotation process enables auditing data quality. This provides transparency when deploying AI models in real-world settings.
Overall, data labeling transforms raw information into structured training data that unlocks the full potential of medical AI. Performing this crucial step rigorously paves the way for developing LLM-based systems that clinicians can trust and effectively use to improve healthcare.
Contact Sapien to Learn More About Our Data Labeling Services for LLMs
Sapien offers end-to-end data labeling services to enable the development of specialized, high-performance LLMs. Our team of global subject matter experts and data labelers meticulously labels datasets tailored to your model's specific domains and use cases.
We implement advanced quality assurance protocols and annotation methodologies to deliver the precise, unbiased data foundation needed to optimize your LLM. Our frictionless integrations and transparent workflows ensure efficiency and maintain complete data provenance.
To learn more about Sapien's data labeling capabilities and how we can accelerate your LLM development, book a demo today.