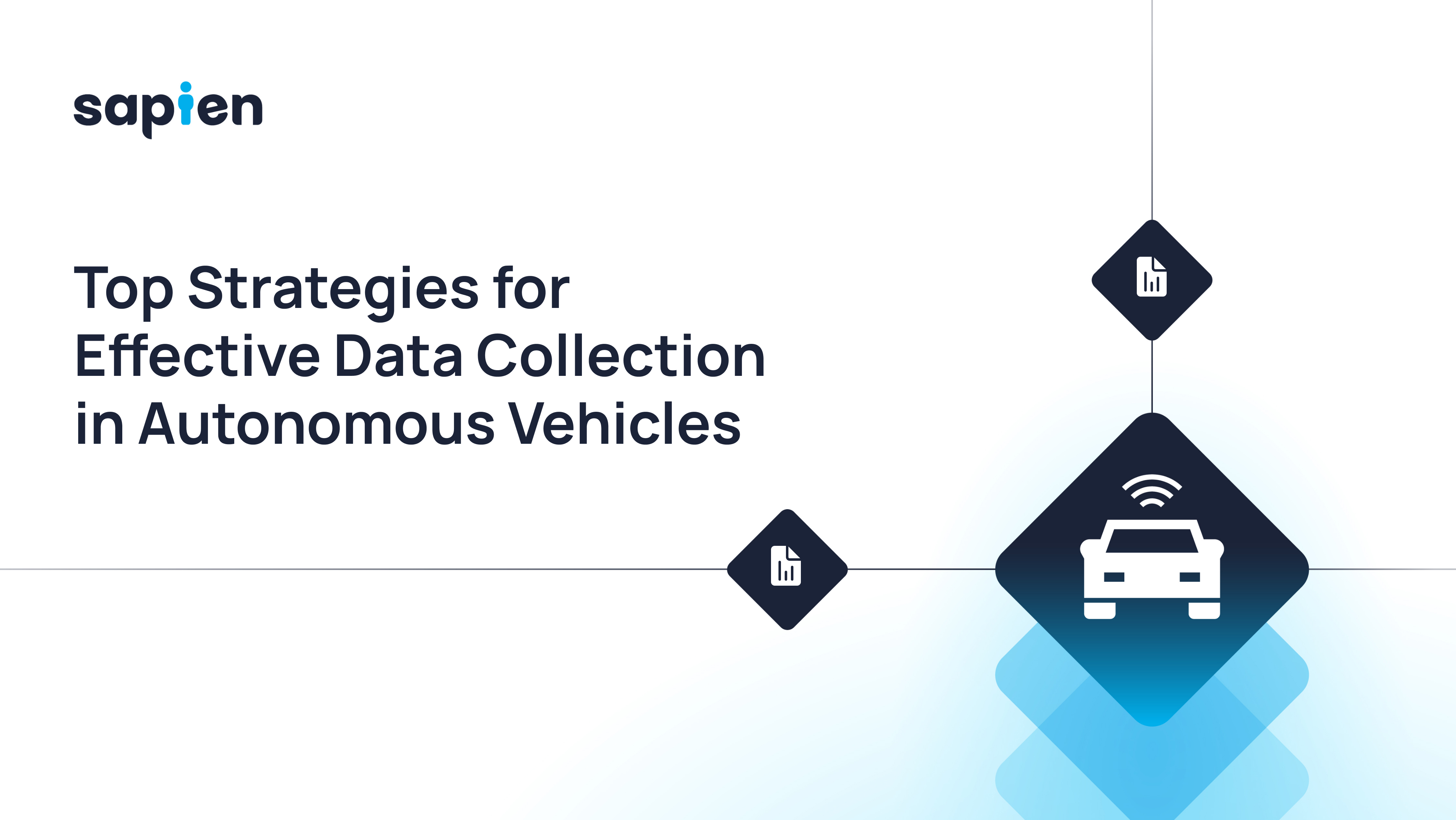
Autonomous vehicles (AVs) are transforming the transportation industry through autonomous vehicle data collection, which is essential for their safe and efficient operation. These vehicles rely on sophisticated systems to sense, process, and respond to real-time environmental changes.
Data collection for autonomous vehicles enhances performance by improving navigation, reducing accident risks, and optimizing driving efficiency. Through continuous data acquisition and analysis, AVs can adapt to varying traffic conditions, detect potential hazards, and make informed driving decisions, ultimately contributing to a safer and more reliable transportation ecosystem.
Key Takeaways
- Foundation of AV Technology: Data serves as the backbone of autonomous vehicle operations, enabling real-time decision-making, navigation, and safety enhancements.
- Types of Data Collected: AVs rely on various data sources, including sensor data, telemetry data, and environmental data to ensure optimal functionality.
- Advanced Processing: AI-driven machine learning algorithms, real-time data processing, and edge computing enhance the efficiency of data utilization.
- Future Trends: The evolution of sensor technologies, AI-based predictive analytics, stricter data regulations, and advancements in cloud and edge computing will define the future of data collection strategies in AVs.
The Role of Data in Autonomous Vehicles
Data is fundamental to AVs, enabling them to perceive surroundings, detect obstacles, and make intelligent driving decisions. Every movement of an AV is based on autonomous vehicle data acquisition and real-time processing. The more high-quality data collected by autonomous vehicles, the better they perform in different environments and traffic conditions.
Autonomous vehicles, equipped with cameras, radar, sonar, lidar, and GPUs, can produce up to 4TB (4,000 GB) of data each day - surpassing the data output of 3,000 conventional vehicles in just one day.
Without robust data collection for autonomous vehicles, AVs would be unable to navigate complex roadways, detect pedestrians, or react to unpredictable situations. Effective data collection is what sets AVs apart from traditional vehicles, allowing them to make informed decisions without human intervention.
As AVs continue to improve through real-time data processing and sensor integration, they become more adept at handling a variety of driving conditions. This evolution contributes significantly to their increasing presence on the roads, leading to greater safety and efficiency in transportation.
In terms of market growth, the global autonomous vehicles market has experienced substantial expansion, with revenue increasing from USD 147.5 billion in 2022 to USD 208.0 billion in 2023. This upward trend is projected to continue, reaching USD 282.2 billion in 2024.
Types of Data Collected
To navigate safely, AVs collect multiple types of data, categorized as follows: This data allows AVs to make informed decisions in real-time, adjusting to ever-changing road conditions. By gathering comprehensive information, AVs can anticipate potential hazards and optimize route planning.
The data collected not only enhances vehicle safety but also improves driving efficiency, reducing fuel consumption and travel time. As AV technology advances, the methods of data collection continue to evolve, integrating more sophisticated sensors and computing capabilities to ensure a seamless and safe driving experience.
Sensor Data
AVs utilize a variety of sensors to perceive their environment, including:
By combining autonomous vehicle data acquisition from these different sensors, AVs create an accurate and dynamic representation of their surroundings. Each sensor has its strengths and limitations, which is why data collection for autonomous vehicles relies on high-precision sensor fusion for maximizing safety and accuracy.
Telemetry Data
Telemetry data provides critical insights into vehicle performance. Data collected by autonomous vehicles constantly monitors speed, acceleration, and braking patterns to ensure optimal operation. System diagnostics help detect mechanical issues early, preventing failures before they occur.
Besides, GPS data allows AVs to determine precise locations, ensuring accurate route planning and navigation. By continuously analyzing telemetry data, how autonomous vehicles collect data allows them to adjust driving strategies in response to real-time conditions, improving efficiency and safety.
Data Processing and Analysis
The enormous volume of data collected by AVs must be processed quickly and accurately to ensure seamless operation. Without efficient processing, AVs would struggle to react to dynamic road conditions, leading to potential safety risks.
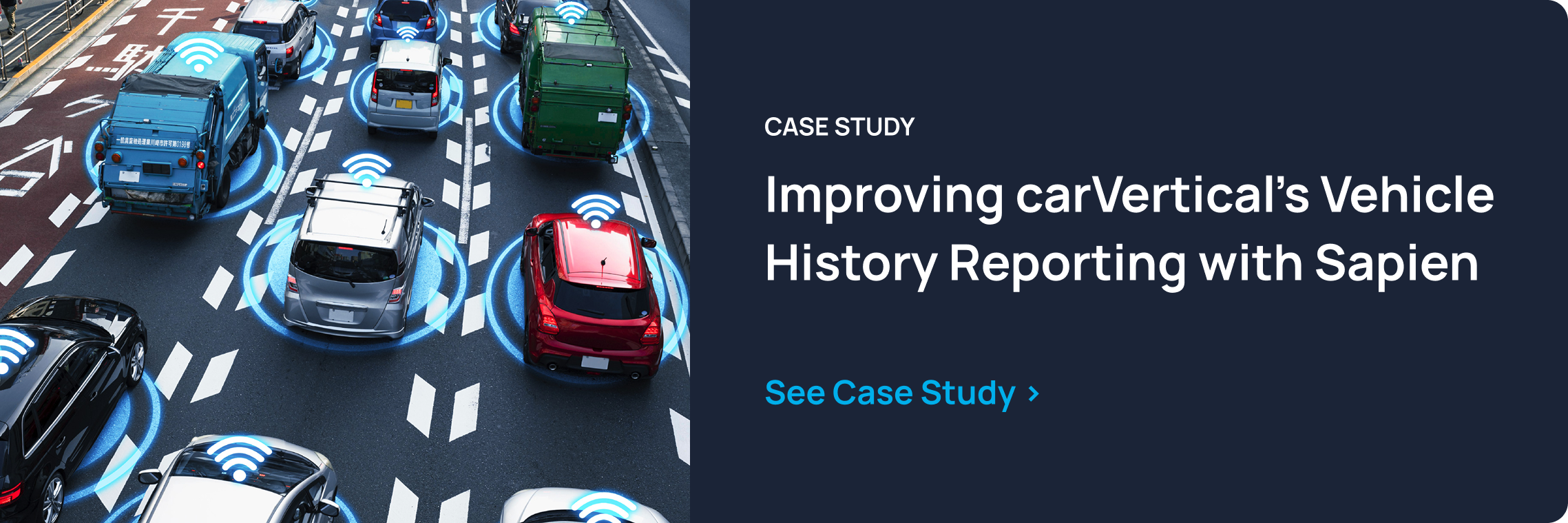
The ability to analyze and interpret data in real-time is crucial for making split-second driving decisions. By leveraging advanced computing techniques, AVs can optimize performance, reduce errors, and continuously improve their self-driving capabilities.
Effective autonomous vehicle data management is key to streamlining these processes, ensuring that data is not only captured but also efficiently organized and accessible for decision-making.
Real-time Data Processing
Data collection is essential for autonomous vehicles to make split-second decisions, such as avoiding obstacles. Autonomous vehicle data collection helps assess road conditions, detect pedestrians, and respond to sudden traffic changes, ensuring safe operation in dynamic environments.
Advancements in LLMs in autonomous vehicles are further enhancing real-time data processing by enabling more sophisticated decision-making. Large language models (LLMs) can process vast amounts of sensor data, improve contextual understanding, and enhance AVs' ability to predict and respond to complex driving scenarios more efficiently. By integrating LLMs, AV systems can achieve greater adaptability and intelligence in unpredictable road conditions.

Machine Learning Algorithms
Machine learning plays a vital role in AV data analysis. By recognizing patterns and predicting behaviors, AVs continuously improve their navigation and object detection capabilities. AI models analyze vast amounts of historical and real-time data to optimize route planning and collision avoidance. Predictive maintenance is another significant advantage, as advanced machine learning can identify potential vehicle malfunctions before they occur, reducing downtime and repair costs. As AI algorithms evolve, AVs will become even more proficient at interpreting complex driving scenarios and enhancing overall safety.
Data Collection Strategies
AV manufacturers employ several strategies to optimize data collection. These strategies ensure that AVs can gather accurate and comprehensive data to enhance their decision-making processes. By using a combination of advanced technologies, manufacturers can improve vehicle perception, safety, and adaptability in diverse driving environments. Continuous advancements in data collection methods also contribute to the overall evolution of autonomous driving capabilities.
Advanced data collection techniques empower AV manufacturers to enhance vehicle perception, safety, and adaptability, driving the evolution of autonomous systems.
Sensor Fusion
Combining multiple sensor inputs enhances AVs' understanding of their surroundings. Sensor fusion merges data from LiDAR, Radar, cameras, and ultrasonic sensors to create a comprehensive environmental model. This approach improves accuracy and reliability by cross-verifying information from different sources. For example, while cameras provide detailed visual recognition, radar excels at detecting objects in poor weather conditions. By leveraging sensor fusion and integrating advanced LiDAR sensors for cars, AVs minimize errors and ensure a more precise perception of the environment.
Edge Computing
Processing data locally within the vehicle rather than sending everything to the cloud improves efficiency. Edge computing enables AVs to make split-second decisions without relying on external servers. This reduces latency, ensuring real-time responsiveness to road conditions. Additionally, edge computing minimizes bandwidth usage by only transmitting essential data to the cloud for long-term analysis. A hybrid approach, combining edge and cloud computing, allows AVs to balance real-time decision-making with extensive data learning and storage.
Data Annotation
Properly labeled data is crucial for training AI models that power AVs. Data labeling for autonomous vehicles involves labeling images, videos, and sensor readings to teach AI algorithms how to recognize objects and behaviors. Manual annotation by human experts ensures high accuracy, but it is time-consuming and expensive. Automated annotation methods, powered by AI, are helping to accelerate the process. However, maintaining high-quality datasets remains a challenge. Without well-annotated data, AVs may struggle to correctly identify objects, leading to potential safety risks.
Challenges in Data Collection
Despite advancements, AV data collection faces key challenges:
- Data Privacy & Security
- AVs collect vast amounts of personal and location data, raising growing privacy concerns.
- Companies must implement robust encryption, anonymization techniques, and strict access controls to protect sensitive information.
- Infrastructure & Connectivity
- Reliable connectivity is essential for AVs to function optimally.
- 5G networks enable fast data transmission, but they are not universally available.
- The lack of standardized data-sharing protocols among different AV brands complicates interoperability.
- Data Labeling and Quality Control
- The accuracy of AI-driven AV systems depends on high-quality labeled data.
- Poorly labeled datasets can lead to incorrect object detection, increasing the risk of accidents.
Transform Autonomous Driving with Smarter Data Solutions
Autonomous vehicle data collection is the foundation of autonomous vehicle technology, enabling safer and smarter transportation. By leveraging autonomous vehicle data acquisition, sensor fusion, machine learning, and edge computing, AVs can process information efficiently and make intelligent driving decisions. As sensor technology, AI, and regulations evolve, how autonomous vehicles collect data will become even more sophisticated, paving the way for fully autonomous mobility.
If you want to stay ahead in this rapidly evolving industry, schedule a consultation with Sapien today to explore cutting-edge data collection solutions tailored to your needs.
FAQs
What is the database for autonomous vehicles?
Autonomous vehicles rely on high-performance databases that store, manage, and process massive amounts of data collected from sensors, cameras, and GPS. These databases support real-time data analysis, machine learning models, and navigation systems, ensuring AVs can operate efficiently and safely.
What AI is used in autonomous vehicles?
Autonomous vehicles utilize artificial intelligence techniques such as machine learning, deep learning, and computer vision. AI-powered models analyze sensor data, recognize objects, predict traffic patterns, and make driving decisions. Neural networks, reinforcement learning, and AI-driven optimization play critical roles in AV functionality.