Data Labeling
Transforming Medicine: AI-Driven Data Collection and the New Standard of Care

AI is transforming healthcare - but its power hinges on one fundamental resource: data. From AI-assisted diagnostics to real-time patient monitoring, every success story in medical AI is rooted in the quality and relevance of the datasets powering these models.
In this article, we explore how AI-driven healthcare data collection is changing the landscape of patient care, and why the future of medicine depends on getting this right.
Key Takeaways
- Data in Healthcare AI: AI in healthcare is powered by high-quality, relevant data. Without it, even the most advanced AI models fail. Accurate health data collection is essential for diagnosing diseases, predicting outcomes, and recommending treatments.
- Types of Data in Healthcare AI: Healthcare data comes in two primary forms - structured (demographics, lab results) and unstructured (clinical notes, medical images).
- Impact of Poor Data: Low-quality or incomplete clinical data collection can lead to significant issues, such as misdiagnoses or missed comorbidities.
- Challenges in Data Collection: Data gathering in healthcare presents technical and ethical challenges, such as maintaining privacy (HIPAA, GDPR) and ensuring data security.
- AI-Driven Data Collection Strategies: AI technologies enable smarter healthcare data collection, including crowdsourcing, web scraping, and hybrid data types (structured and unstructured).
- Advanced AI Algorithms for Data Processing: AI models, particularly deep learning algorithms, help process complex healthcare data, reducing errors and improving decision-making speed.
The Role of Data in Modern Healthcare AI
AI in healthcare isn't theoretical - it's already here. Yet, without quality health data management, its potential collapses.
“You can’t have ethical AI in healthcare without ethical data. If your data is flawed, your AI will be too.” - Dr. Eric Topol, Cardiologist and Author of Deep Medicine
AI systems in healthcare depend on medical data collection to create models for diagnosing diseases, predicting patient outcomes, and recommending treatments. These models analyze patient data to uncover patterns, assess risk factors, and suggest effective interventions.
- Structured data includes patient demographics, medical history, lab results, and other quantifiable data stored in tables.
- Unstructured data includes clinical notes, medical images, and voice recordings, which are more complex and require sophisticated AI models like Natural Language Processing (NLP) and image recognition.
For AI to be effective in healthcare, the data it processes must be of high quality. This includes accurate and representative datasets that reflect the complexity of medical conditions, treatments, and patient demographics.
A McKinsey & Company report found that over 50% of AI failures in healthcare are due to poor data quality or inconsistency. AI tools learn from health data analysis - so incomplete, biased, or irrelevant datasets can create dangerous models.
Real-World Impact of Poor Data
Here are concrete examples of how specific data issues directly affect AI performance and patient well-being:
Key Healthcare Applications Powered by AI Data
AI in healthcare is only as powerful as the data it is trained on. With rich, domain-specific resources - such as high-quality image datasets - AI can transform critical areas of patient care. Here are some of the most impactful applications:
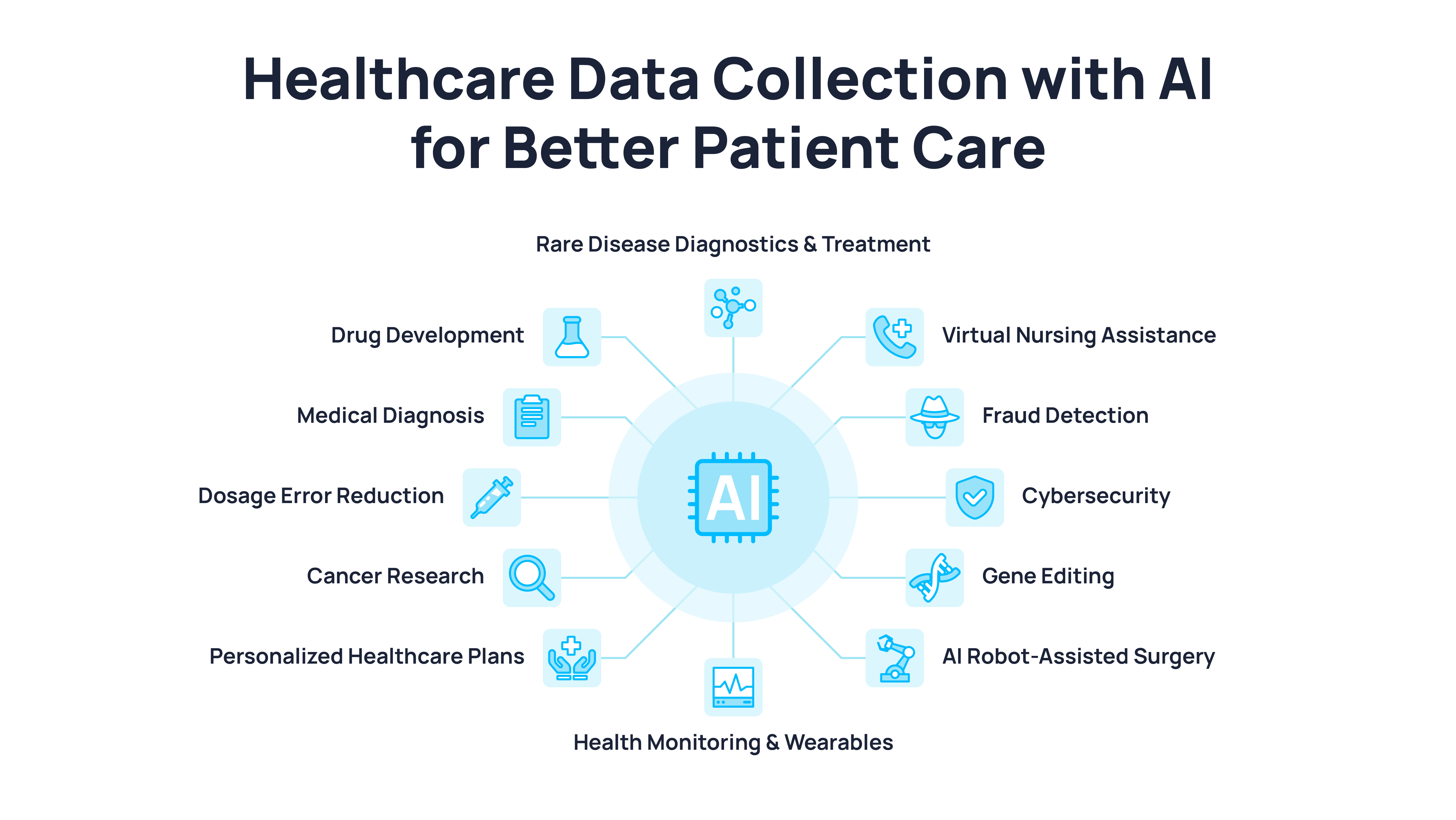
Medical Imaging and Diagnostics
AI has transformed medical imaging, enabling faster and more accurate diagnoses. Key advancements include:
- AI-Enhanced Diagnostics: AI algorithms analyze medical images (X-rays, CT scans, MRIs) to detect abnormalities such as tumors, fractures, or infections.
- Early Detection: AI models can spot subtle changes in images that human doctors might miss, improving early diagnosis of diseases like cancer and heart disease.
One notable success story is Google’s DeepMind, which developed an AI system capable of diagnosing over 50 different eye conditions from retinal scans. The model achieved diagnostic performance on par with leading ophthalmologists, demonstrating how AI can complement medical expertise and expand diagnostic capacity.
Natural Language Processing for Clinical Notes
NLP in healthcare is used to convert unstructured text data (such as doctor-patient notes and pathology reports) into structured data, making it actionable for AI systems.
- Improved Decision Making: NLP systems assist healthcare providers in making smarter, real-time decisions by analyzing unstructured clinical data.
- Clinical Notes Analysis: By processing patient records, AI can flag critical information, predict complications, and recommend next steps.
Voice and Speech Recognition in Healthcare
AI-powered voice recognition technologies are becoming increasingly important in healthcare settings. They allow for hands-free documentation and help streamline patient-provider interactions.
- Telemedicine Integration: AI enables remote consultations, voice commands, and transcription of clinical notes, improving healthcare accessibility.
- Multilingual Support: Voice recognition technology enables the collection of data from diverse linguistic populations, ensuring inclusive healthcare services.
Challenges in Healthcare Data Collection
Collecting healthcare data for AI isn’t as straightforward as it seems. There are technical, ethical, and operational barriers that must be addressed for AI to truly deliver value.
Privacy and Compliance
Maintaining privacy and adhering to global standards such as HIPAA, General Data Protection Regulation (GDPR), and other data protection laws is a significant challenge in health data collection methods.
Lack of Domain Expertise
Healthcare data is highly specialized. General data labelers often struggle with medical terminology, which can lead to inaccurate annotations.
- Specialized Annotation: Medical experts must be involved in the annotation process to ensure accuracy.
- Collaboration: AI teams must work closely with healthcare professionals to interpret and label data accurately.
Annotation at Scale
Manually annotating medical datasets is time-consuming and costly. Scaling these efforts while maintaining high quality is challenging, but AI-powered tools can help automate the process.
- Automated Data Annotation: AI tools can assist with the initial labeling, but human experts are still required for quality control.
AI-Driven Healthcare Data Collection Strategies
To overcome these challenges, leading organizations are turning to smarter, AI-augmented data collection strategies for collecting and refining healthcare data.
Smart Collection Techniques
To gather large volumes of diverse healthcare data, AI-driven collection techniques include:
- Crowdsourcing: Gathering patient feedback through surveys or crowd-based platforms.
- Web Scraping: Extracting publicly available health data from trusted sources for analysis.
- Hybrid Data Types: Collecting both structured (tabular) and unstructured (text, voice, images) data to build comprehensive models.
Multi-Stage Validation & Quality Control
Ensuring the accuracy of healthcare data requires multiple stages of validation:
- Automated QA Tools: These tools assess data quality by checking for consistency and completeness.
- Expert Review: Medical professionals verify the data, ensuring its reliability and relevance.
Using Advanced AI Algorithms for Data Processing
AI algorithms, especially deep learning models, are used to process and extract insights from complex healthcare data types. These models help:
- Reduce Errors: Minimizing human error in data processing.
- Improve Efficiency: Enhancing the speed at which data is processed, enabling faster decisions.
AI-Driven Healthcare with Smarter Data Collection
AI is transforming healthcare, but its success hinges on high-quality data. From diagnostics and clinical notes to voice recognition and real-time monitoring, every breakthrough relies on accurate, diverse, and well-annotated datasets. As the article shows, poor data can lead to serious risks - from misdiagnoses to biased outcomes - while reliable data empowers faster, smarter, and more inclusive care.
That’s where Sapien comes in. We specialize in healthcare-grade data collection and annotation, combining expert oversight with scalable AI tools. Whether you need structured EMR data, annotated medical images, or multilingual voice samples, Sapien helps you build AI systems that are safe, ethical, and ready to make a real impact. Contact us today to power your next healthcare innovation with data you can trust.
FAQs
What impact does AI have on reducing healthcare costs?
AI can help reduce healthcare costs by improving diagnostic accuracy, optimizing resource allocation, and enhancing operational efficiency in healthcare facilities, ultimately leading to fewer misdiagnoses, unnecessary treatments, and hospital readmissions.
How does AI ensure accuracy in diagnosing rare diseases?
AI can improve diagnostic accuracy for rare diseases by analyzing large datasets of patient records and medical literature, identifying patterns that might not be immediately obvious to human doctors, and offering a second opinion or early warning.
Can AI help with mental health diagnoses and treatments?
AI can aid in mental health by analyzing patient data such as behavioral patterns, speech, and clinical notes to detect early signs of mental health disorders and recommend treatment options.