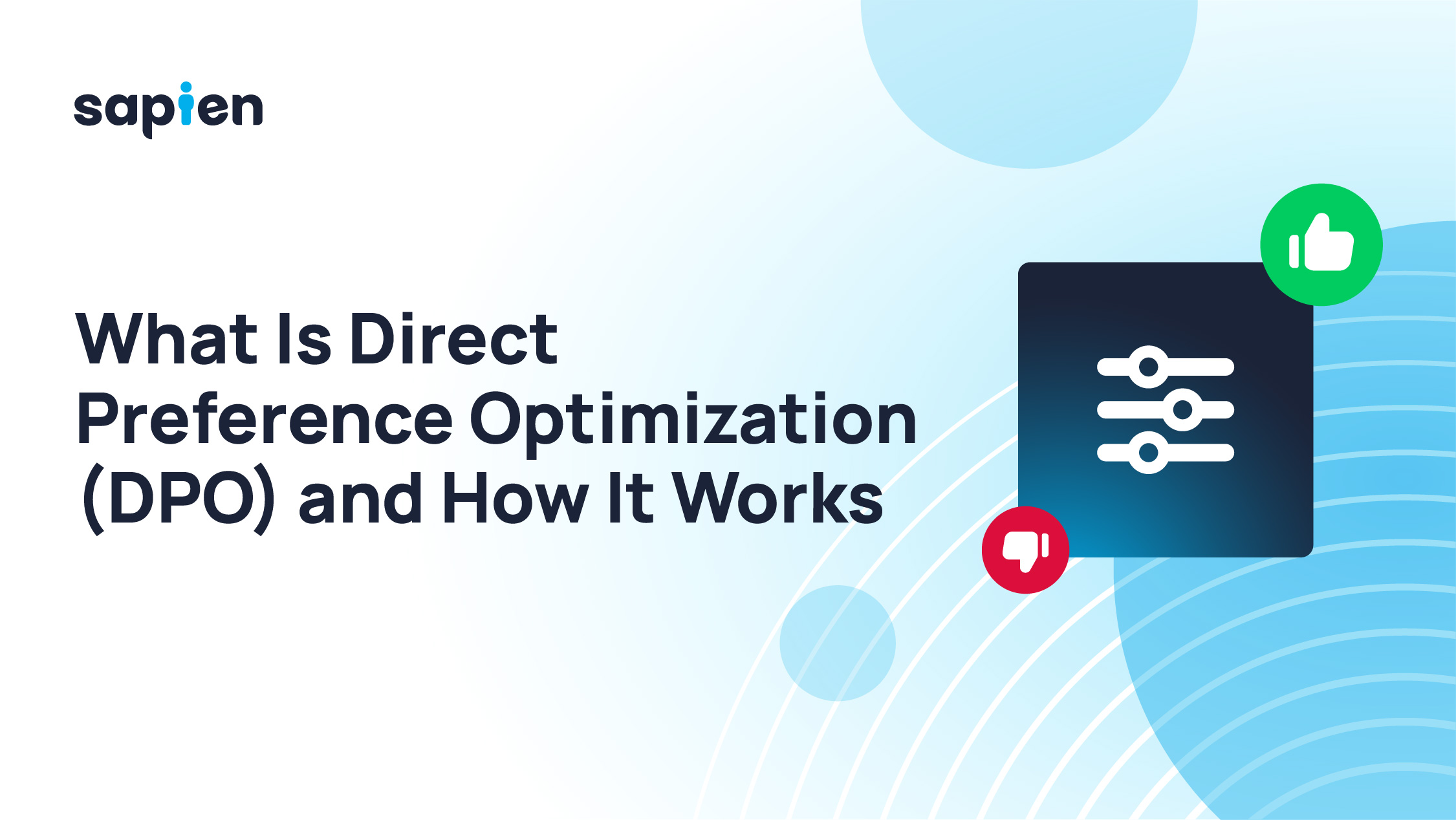
In today's fast-paced digital world, businesses and industries are constantly seeking new ways to optimize their operations, enhance customer experiences, and make smarter decisions. Direct Preference Optimization (DPO) is one such technique that allows companies to tailor their strategies based on real-time consumer preferences.
This powerful tool not only helps improve marketing efforts but also drives more accurate decision-making in sectors such as e-commerce, machine learning, and customer experience. In this article, we’ll explore what DPO is, how it works, its applications, and how businesses can leverage it to optimize their processes and drive success.
Key Takeaways
- Direct Preference Optimization (DPO) tailors strategies based on real-time user feedback.
- It enables more personalized experiences and enhances decision-making.
- DPO is particularly useful in industries like marketing, e-commerce, and machine learning.
- The process involves collecting and analyzing user data, modeling preferences, and continuous optimization.
- DPO algorithm is central to refining decision-making through iterative feedback.
What is Direct Preference Optimization (DPO)?
Direct Preference Optimization (DPO) is an advanced optimization technique that focuses on understanding and adjusting processes based on user preferences and feedback. Unlike traditional methods, which typically rely on set rules or generalized data, DPO uses real-time user input to make decisions and optimize processes dynamically.
The DPO algorithm plays a crucial role in helping businesses continuously refine their strategies based on user behavior. It is designed to adapt to changing preferences and provide optimal solutions through feedback loops that are rooted in direct user interactions.
Relationship Between DPO and Traditional Optimization Methods
Traditional optimization methods often involve predefined variables and decision rules to enhance outcomes, such as maximizing profit or minimizing costs. However, these methods can fall short when dealing with complex, ever-changing consumer preferences or real-time feedback. In contrast, DPO adapts based on user-specific inputs and iteratively refines strategies to ensure they meet the dynamic nature of customer needs.
Comparison with Other Optimization Techniques
While DPO is powerful, it’s important to compare it with other techniques like Direct Policy Optimization, Reinforcement Learning (RL), and Genetic Algorithms (GA), both of which are also used for optimization tasks.
DPO generally stands out for its ability to implement changes in real-time based on direct user interactions, making it an attractive option for businesses that require dynamic adaptation.
How Does Direct Preference Optimization Work?
At its core, DPO works by continuously collecting data on consumer preferences-whether through clicks, ratings, or purchases-and using this data to adjust the optimization model. This feedback loop ensures that the system is always aligned with the user’s evolving needs and preferences.
Role of User Preferences and Feedback in Optimization
User feedback is integral to DPO. By constantly gathering information on what users like and don’t like, businesses can tailor their products, services, and experiences. This helps in better aligning the decision-making process with actual user desires rather than relying on generalized assumptions.
Step-by-Step Process of DPO in Practice
- Collecting User Data: The first step is to gather data about user preferences through various means like clicks, surveys, purchases, and interactions. This data can come from multiple sources, such as websites, apps, or social media platforms.
- Modeling Preferences and Decision Criteria: Once data is collected, it is analyzed and modeled to understand the preferences and patterns of different customer segments. These models predict how users will behave in future interactions.
- Optimization Process: Based on the models, the system adjusts its parameters to optimize outcomes-whether it’s recommending the right product, offering personalized pricing, or adjusting a marketing message.
- Iteration and Continuous Improvement: The process doesn’t stop at optimization. DPO continuously learns from new user data and feedback to refine its predictions and strategies, ensuring that the system becomes more accurate over time.
Applications of DPO
Marketing and Customer Experience
- Personalization of Recommendations: By understanding individual preferences, DPO allows businesses to recommend products or services that are more likely to meet the customer’s needs. This personalized approach leads to higher conversion rates and greater customer satisfaction.
- Tailored Product Suggestions: With real-time feedback, businesses can dynamically suggest products to users based on their current interests, increasing the likelihood of a sale.
E-commerce
- Price Optimization Based on Customer Preferences: DPO helps e-commerce platforms set dynamic prices by taking into account what users are willing to pay for specific products, thereby maximizing profits without alienating customers.
Machine Learning and AI
- Enhancing Decision-Making Models in AI: DPO can significantly improve decision-making models by continuously feeding them with user preference data, ensuring the system always produces the most relevant and accurate predictions.
Other Industries
- Healthcare: DPO can be used to personalize treatment recommendations based on patient preferences, improving engagement and health outcomes.
- Entertainment: Streaming services can use DPO to suggest content based on user behavior, keeping audiences engaged for longer periods.
- Finance: Banks and financial services can optimize their offerings based on customer feedback, providing more tailored investment products.
Advantages of DPO
- Increased Accuracy in Predicting Consumer Choices: DPO allows businesses to make data-driven decisions that reflect the true preferences of their customers, leading to more accurate predictions.
- Enhanced User Satisfaction and Engagement: By aligning with customer needs, DPO boosts satisfaction, engagement, and loyalty, which translates into higher retention rates.
- Better Alignment with Customer Needs: The iterative nature of DPO ensures that customer preferences are continuously met, enhancing overall experience and satisfaction.
- Efficiency in Resource Allocation and Process Optimization: By focusing on user-driven decisions, businesses can optimize their resources more effectively, minimizing waste and maximizing value.
Challenges and Limitations
- Data Quality and Collection Challenges: To be effective, DPO requires high-quality data. Poor or incomplete data can lead to suboptimal results.
- Potential Bias in Preference Modeling: Bias in the data collection process or modeling techniques can skew the optimization process, leading to unfair or inaccurate results.
- Computational Complexity and Scalability: For large-scale applications, the process of continuously collecting, modeling, and optimizing can become computationally expensive and complex.
How to Choose Between DPO and RLHF
When deciding between DPO and RLHF, it's essential to understand the specific needs of your business and the type of decision-making process you're aiming to enhance.
- DPO is best suited for scenarios where you need real-time feedback directly from user preferences to personalize experiences or optimize products and services. It's ideal when you want continuous improvement based on direct consumer behavior and interaction.
- RLHF, on the other hand, is more appropriate for situations where a machine learning model must be trained with human feedback to refine its actions over time, especially when there are complex decision-making processes involved, such as autonomous systems or gaming environments.
Future of Direct Preference Optimization
- Trends in DPO Research and Development: As AI and machine learning evolve, DPO will become even more refined, allowing for deeper insights into consumer behavior.
- How AI and Big Data Are Shaping the Future of DPO: The continuous evolution of AI and big data analytics will make DPO more accessible and efficient, enabling businesses to optimize in real-time and at scale.
- Potential Use Cases and Emerging Industries for DPO: DPO will likely expand into industries like autonomous vehicles, personalized health care, and urban planning, where user preferences play a central role in decision-making.
Why Choose Sapien for Direct Preference Optimization?
By leveraging advanced algorithms, including the DPO algorithm, and real-time customer data, Sapien helps companies create personalized experiences, optimize marketing strategies, and improve decision-making across various industries. With Sapien, businesses can harness the power of Direct Policy Optimization to stay ahead of the competition, offering tailored solutions that resonate with their customers’ preferences. Whether you're looking to enhance your e-commerce strategy, improve customer satisfaction, or optimize your AI models, Sapien is the platform you need to take your optimization efforts to the next level.
FAQ
How does DPO improve user engagement?
By continuously adapting to real-time user feedback, DPO personalizes experiences, making recommendations and content more relevant to individual preferences, which boosts user engagement and satisfaction.
What data is most important for effective Direct Preference Optimization?
To implement DPO successfully, high-quality data such as user clicks, ratings, preferences, purchase behavior, and interaction history is essential for building accurate predictive models and ensuring optimal decision-making.
How can DPO be used to improve customer retention?
By delivering personalized experiences and recommendations based on real-time feedback, DPO helps build stronger connections with customers, increasing satisfaction and loyalty, which ultimately leads to higher retention rates.