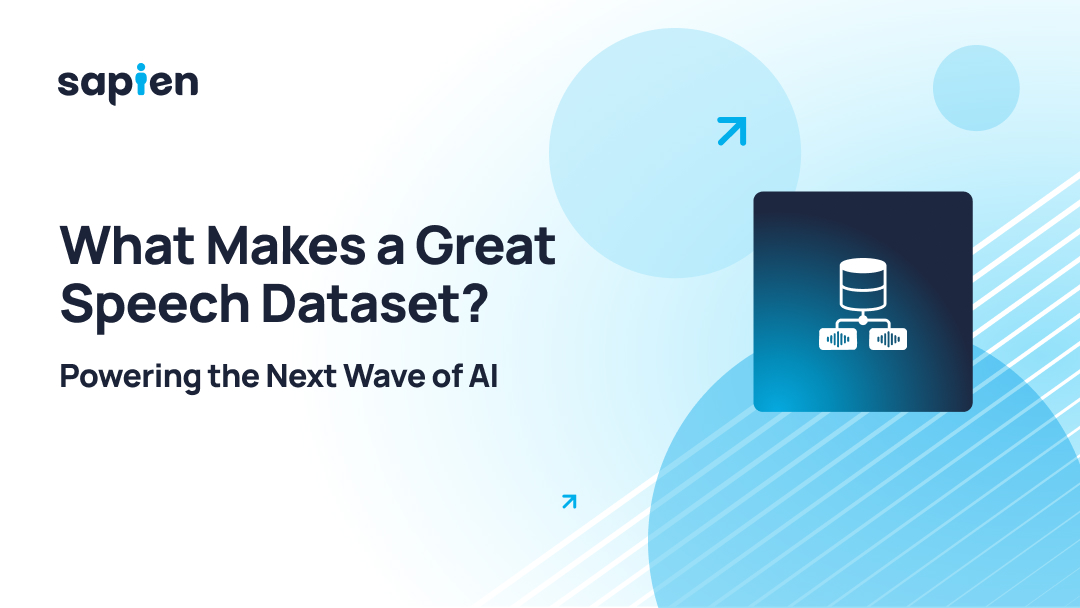
Whether it's powering smart assistants, enhancing accessibility tools, or improving real-time transcription services, the need for high-quality, diverse, and well-annotated datasets for speech recognition has never been more critical.
Speech recognition databases are not just essential for Automatic Speech Recognition (ASR) systems but are also crucial for training advanced voice technologies and enhancing AI applications. Unlocking the full potential of speech recognition datasets opens up a future where human-machine interaction becomes seamless, accessible, and truly global.
Key Takeaways
- Speech Dataset Quality: High-quality, diverse, and balanced speech datasets are essential for building reliable AI applications, minimizing bias, and ensuring broader accessibility.
- Speech Recognition and Synthesis Technologies: Success in both Automatic Speech Recognition (ASR) and Text-to-Speech (TTS) depends on training with diverse, clearly annotated, and domain-specific datasets.
- Challenges in Data Collection: Gathering high-quality speech data is complex due to privacy concerns, noise interference, demographic underrepresentation, and scalability issues.
Understanding Speech Recognition and Synthesis
Speech technologies are no longer futuristic concepts; they are integral parts of our daily lives, from smart assistants to customer service bots. In fact, a 2024 report by MarketsandMarkets projects that the speech and voice recognition market will grow to USD 28.1 billion by 2027, driven by the explosion of AI-based communication systems across sectors.
This massive growth shows the urgent need for a deeper understanding of two foundational technologies: Speech Recognition and Speech Synthesis.
What is Speech Recognition?
Speech recognition involves translating spoken language into written text. This process relies heavily on Automatic Speech Recognition (ASR) technologies integrated with Natural Language Processing (NLP). It enables machines to "listen" and respond intelligently to human input.
What is Speech Synthesis?
Speech synthesis, commonly referred to as Text-to-Speech (TTS), focuses on creating human-like voices from written input. With innovations like neural voice cloning, synthesized speech today can mimic real individuals' intonations, pacing, and emotional tones, making AI interactions feel more natural.
What Makes a High-Quality Speech Dataset?
High-quality audio dataset are crucial for the success of both speech recognition and synthesis models. These datasets fuel a variety of AI-driven applications, from speech-to-text systems to voice-enabled devices. Here's what makes a dataset for voice recognition truly effective:
"Without large, diverse, and high-quality datasets, even the most sophisticated AI models fall short in real-world performance." Dr. Andrew Ng, AI Pioneer
Challenges in Collecting Speech Data
Despite its importance, building speech recognition databases poses major challenges:
- Privacy Concerns: Consent, anonymization, and ethical sourcing are critical.
- Noise and Distortion: Real-world environments often degrade data quality.
- Lack of Diversity: Overrepresentation of certain accents or demographics introduces bias.
- Cost and Scalability: Large-scale, high-quality data collection remains prohibitively expensive for many.
A 2023 study by Stanford University found that models trained on homogeneous datasets performed up to 35% worse when exposed to diverse real-world conditions compared to those trained on diverse datasets.
These barriers limit access to truly representative and usable speech datasets, especially for smaller AI companies and startups.
How Sapien Solves These Challenges
Faced with these complex challenges, organizations must seek innovative partners capable of delivering high-quality datasets for AI, especially speech data, at scale. Traditional methods often fall short - either limited by rigid infrastructures or burdened by prohibitive costs. This gap creates an urgent need for modern, flexible, and highly specialized solutions that ensure robust and diverse datasets for AI training in speech recognition and related applications.
Sapien's innovative approach directly addresses these pain points:
- Multilingual Audio Collection: Diverse global network (contributors from 103+ countries).
- Gamified Engagement: Blockchain-based rewards increase labeler participation and quality.
- Advanced QA Systems: Integrated Human-in-the-Loop (HITL) and automated quality assurance.
- Custom Solutions: Tailored audio data for industries like healthcare, autonomous vehicles, and edtech.
Using their decentralized workforce and custom QA processes, Sapien’s advanced data collection service delivers thousands of high-quality, diverse audio recordings at scale. This enables clients to achieve state-of-the-art transcription accuracy across a wide range of languages and accents.
Unlocking the Future with Better Speech Datasets
Investing in curated speech datasets today means building the foundation for inclusive, efficient, and groundbreaking AI applications tomorrow. Organizations that prioritize diversity, clarity, and precision in their data sourcing will not just keep pace with innovation - they will define it.
High-quality, diverse speech datasets are not just enablers; they are accelerators of AI innovation. By investing in better speech data, companies can:
- Develop more accurate and inclusive speech recognition and synthesis models.
- Expand into new global markets by supporting multilingual, multicultural AI interactions.
- Enable cutting-edge innovations in LLMs, accessibility, and customer engagement platforms.
If you're ready to elevate your AI projects with diverse, high-quality speech datasets, partner with Sapien.io. Tap into our scalable, decentralized workforce and cutting-edge QA systems to power the next generation of AI.
Contact Sapien.io today to discuss custom solutions that fit your exact needs.
FAQs
How do you evaluate the quality of a speech dataset?
Dataset quality can be measured using metrics like Word Error Rate (WER), Signal-to-Noise Ratio (SNR), and phoneme error rates. Human review is also a critical part of quality evaluation.
What is the difference between natural and synthetic speech data?
Natural speech data is collected from real human speakers, while synthetic speech is generated by Text-to-Speech (TTS) systems. Training models on natural data usually results in higher authenticity, but synthetic data can augment datasets for specific scenarios.
What industries benefit most from high-quality speech datasets?
Industries like healthcare, finance, education, automotive, and entertainment heavily rely on precise speech datasets for applications like virtual consultations, fraud detection, and interactive learning.
Can synthetic voices be used to create speech datasets?
Yes, synthetic voices are often used to expand datasets or simulate rare accents and scenarios. However, they should complement, not replace, real human speech data for best results.