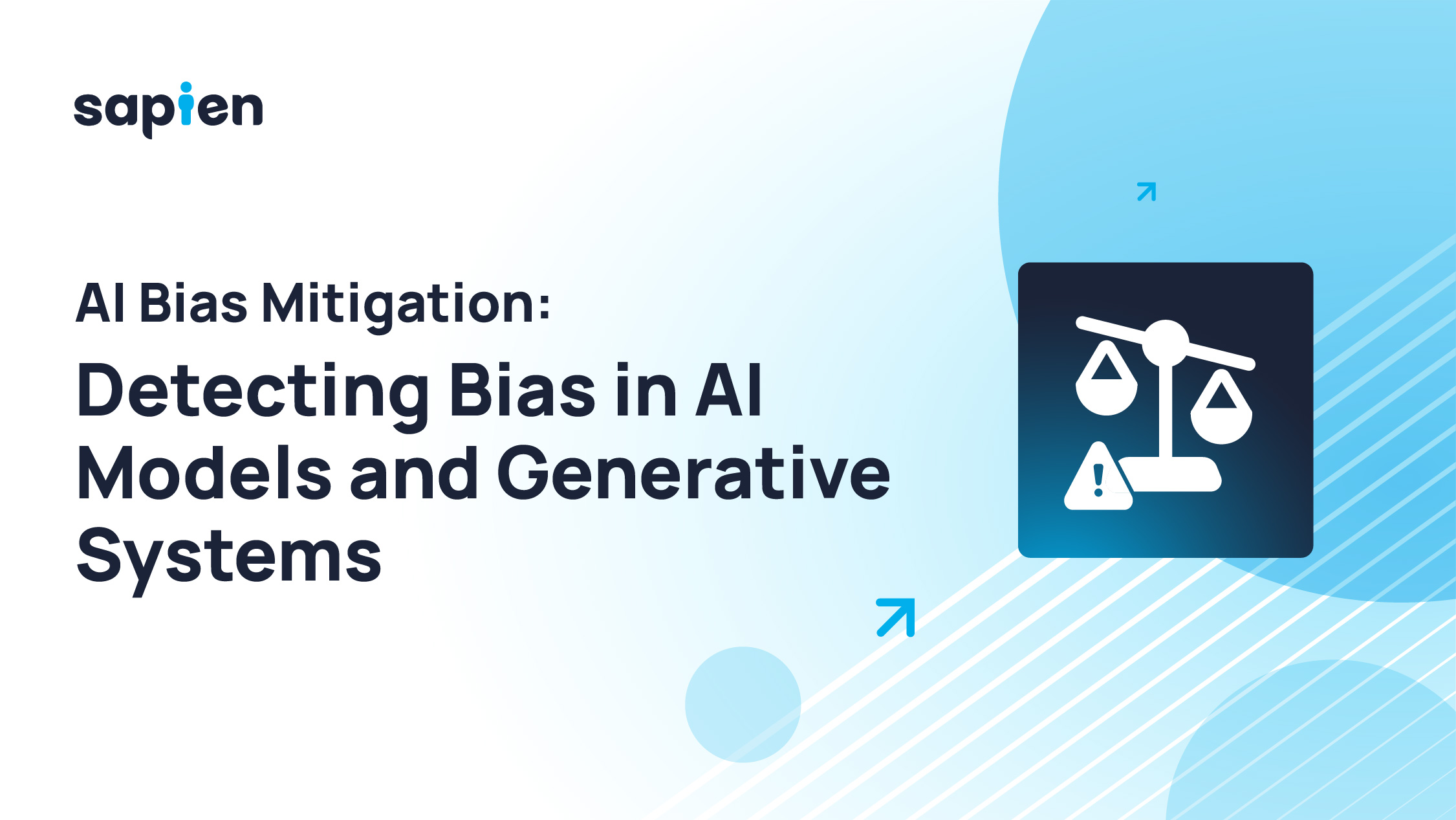
Artificial Intelligence (AI) has revolutionized industries across the globe, from healthcare and finance to retail and entertainment. As generative systems like large language models (LLMs) and other AI tools continue to shape our world, the issue of AI bias has become increasingly significant. AI systems, if not carefully designed and monitored, can perpetuate and even amplify existing biases in society. This article explores AI bias detection and mitigation strategies, highlighting the importance of addressing bias in AI models and generative systems.
Key Takeaways
- Origins of AI Bias: Bias in AI systems can stem from imbalanced training data, flawed algorithm design, or unconscious human influence during development.
- Types of Bias in AI Models: AI bias generally falls into three categories - data bias, algorithmic bias, and societal bias - each with distinct implications for fairness and equity.
- Consequences of Bias: Unchecked bias can lead to real-world harm, including discrimination in hiring, lending, law enforcement, and access to services.
- Methods for Detecting Bias: Bias can be identified using statistical tools, fairness audits, and performance testing across different demographic groups.
- Strategies for Mitigation: Effective bias mitigation involves pre-processing diverse data, incorporating fairness constraints during model training, and adjusting outputs post-deployment.
Understanding AI Bias
Before diving into how to mitigate AI bias, it’s essential to understand what AI bias is and where it originates.
Definition of AI Bias and Its Origins
AI bias refers to the systematic favoritism or discrimination introduced by artificial intelligence systems that result from biased data, algorithms, or human influence during the AI model development process. These biases can arise at various stages, from training datasets to algorithmic design, and can perpetuate harmful stereotypes or unfair treatment.
The primary sources of AI bias include:
- Data Bias: If the data used to train an AI model is unrepresentative or reflects societal biases, the model will learn and reproduce these biases. For example, if a hiring algorithm is trained predominantly on resumes from one demographic group, it may favor candidates from that group.
- Algorithmic Bias: Even if the data is neutral, algorithms can still be biased if they are designed in a way that inherently favors certain outcomes or groups. The design of an AI model, including the choice of features and decision-making processes, can introduce bias.
- Human Bias: AI models are often developed by humans, and as such, they can inherit human biases. Developers' unconscious biases during model creation or data labeling can influence the system’s behavior.
To learn more about AI data labeling check out our in-depth article.
Types of AI Bias
There are several types of AI bias, each with unique implications for society:
Real-World Examples of AI Bias and Its Consequences
AI bias can have severe consequences in various sectors. For instance:
- Discrimination in Hiring: Recruitment tools used by companies have been found to discriminate against female candidates due to biased training data that predominantly features male resumes.
- Facial Recognition Issues: Several facial recognition systems have been shown to have higher error rates for people of color and women, leading to wrongful arrests or identification failures.
- Credit Scoring: AI models used in lending have been known to deny loans to minority groups due to biased historical financial data.
Why AI Bias is a Problem
AI bias poses several serious challenges, both from an ethical and societal perspective. These challenges can impact businesses, individuals, and entire communities.
Ethical Concerns: Fairness, Accountability, and Transparency
The most critical ethical issue surrounding AI bias is fairness. If AI systems perpetuate existing social inequalities, they can lead to unfair treatment of marginalized groups. Ensuring accountability for AI decisions and maintaining transparency in AI processes are fundamental for fostering trust in AI technologies.
Legal and Social Implications
The consequences of AI bias extend beyond ethical concerns. Biased AI systems can lead to discriminatory practices, which violate civil rights laws. This can result in lawsuits, financial penalties, and damage to a company’s reputation.
Additionally, AI bias can foster mistrust in AI systems, leading to resistance from the public and regulatory bodies.
Impact on Business and Society
AI bias can also negatively affect business operations. Companies that fail to address bias may experience reduced customer trust, legal disputes, and damaged brand reputation. On a societal level, AI bias contributes to inequality and social harm, further entrenching stereotypes and marginalizing disadvantaged communities.
Detecting Bias in AI Models
Detecting AI bias is the first step in mitigating its harmful effects. Without detecting bias early in the AI development process, companies cannot ensure the fairness of their models.
Importance of Bias Detection in AI Development
Bias detection allows developers to identify where and how bias enters the system. The earlier this process is carried out, the easier it is to adjust the model and the data to minimize negative impacts.
Methods for Detecting AI Bias
There are several methods for detecting bias in AI models:
Mitigating AI Bias
Once bias is detected, the next step is mitigation. Several strategies can be implemented to reduce bias in AI models.
Strategies for Reducing Bias in AI Models
So, reducing bias in AI requires a multifaceted approach across different stages of the model development lifecycle. From improving the quality of training data to adjusting algorithms and refining model outputs, each step plays a crucial role in promoting fairness and inclusivity. Here are some strategies aim to minimize both visible and hidden biases while maintaining model effectiveness.
Pre-processing: Ensuring Diverse and Representative Data
This involves modifying the training data to include more diverse and representative samples, ensuring that no group is underrepresented.
In-processing: Adjusting Algorithms to Promote Fairness
Modifying algorithms to explicitly incorporate fairness objectives can reduce bias. For instance, fairness constraints can be added to decision-making processes during model training.
Post-processing: Modifying Outputs to Reduce Bias
Once the AI model has been trained, the outputs can be adjusted to ensure fairness. This may involve recalibrating predictions or decisions to account for biases detected in the initial outputs.
Importance of Diverse Teams and Perspectives in AI Development
A diverse team brings varied perspectives and life experiences, which can help in identifying and addressing biases that a homogeneous team might overlook. Diversity in teams contributes to building more balanced and fair AI systems.
Ethical Frameworks and Guidelines for AI Development
Adopting ethical frameworks is critical for AI fairness. Guidelines such as the IEEE Standards for AI, EU AI Ethics Guidelines, and other ethical principles provide a structured approach to mitigating bias during development.
Challenges in Mitigating AI Bias
While mitigating bias is crucial, it is not without its challenges.
Technical Challenges
Balancing fairness and accuracy is a complex task. In some cases, improving fairness might slightly reduce model performance. Developers need to make careful trade-offs to ensure that the AI system remains efficient while being fair.
Social Challenges
Addressing societal biases embedded in data is difficult. These biases are often deep-rooted and can be challenging to eliminate entirely, especially when data is collected from historical systems that reflect past inequalities.
Trade-offs Between Fairness, Transparency, and Performance
While AI fairness is essential, there are often trade-offs with transparency and performance. Striking the right balance is key, as overly complex models might not be transparent, and overly simplistic ones may lack the performance needed for critical tasks.
Future Directions and Emerging Trends
As AI continues to evolve, several emerging trends are shaping the future of AI bias mitigation.
Advancements in AI Fairness Research
New research is constantly pushing the boundaries of AI fairness, with improved algorithms and tools for detecting and mitigating bias in AI systems. The use of counterfactual fairness, adversarial debiasing, and other cutting-edge techniques are expected to lead to more equitable AI solutions.
Role of Regulation and Legislation
Governments and regulatory bodies are beginning to take action to mitigate AI bias through regulation. Expect to see more legislation requiring companies to test their AI systems for fairness and address any detected biases.
Integration of Ethical AI Practices in the AI Model Lifecycle
Ethical AI practices are increasingly being integrated into every phase of the AI lifecycle, from data collection and model development to deployment and ongoing monitoring. This proactive approach ensures fairness is maintained throughout.
How Sapien is Leading the Way in AI Bias Mitigation
Sapien is committed to developing AI solutions that not only perform well but also remain ethical and fair. By leveraging state-of-the-art tools and frameworks for detecting and mitigating bias, Sapien ensures that its AI models are built on fair and representative data.
Sapien’s Commitment to Ethical AI
Sapien’s core mission is to ensure that AI systems are used responsibly and fairly. We take pride in building systems that reduce bias, promote inclusivity, and contribute positively to society.
Innovative Solutions for Bias-Free AI
Our platform incorporates AI bias detection tools and fairness metrics, ensuring that every AI solution we provide is free from harmful biases. We work closely with clients to develop solutions tailored to their needs, using the latest advancements in AI fairness research.
Empowering Businesses with Ethical AI
Sapien empowers businesses to implement AI tools that are not only efficient but also ethical. By working with us, companies can create AI systems that promote fairness, reduce discrimination, and enhance trust among their users.
FAQ
How can businesses ensure fairness in AI without sacrificing performance?
Businesses can achieve fairness without sacrificing performance by adopting techniques such as fairness-aware machine learning algorithms that optimize both fairness and accuracy, ensuring that the AI model remains effective while promoting equal treatment.
Can AI bias be mitigated in generative systems like language models?
Yes, bias in generative AI models can be mitigated by using diverse training datasets, incorporating fairness constraints during model development, and employing techniques like adversarial debiasing to adjust the model's output.
How do Sapien's AI tools help businesses address bias in their models?
Sapien’s AI platform integrates bias detection tools and fairness metrics throughout the development process, ensuring that businesses can detect, monitor, and mitigate biases, creating AI systems that are both effective and fair.