
Geospatial data is transforming industries worldwide by enhancing decision-making processes, optimizing operations, and enabling deeper insights into various sectors. In today’s technological landscape, geospatial data plays a pivotal role in sectors ranging from urban planning to healthcare. This article will dive deep into what geospatial data is, its various collection methods, applications across industries, and the challenges faced when working with it.
Key Takeaways
- What Is Geospatial Data: Geospatial data links information to specific locations on Earth, helping to analyze and visualize spatial relationships.
- Types of Geospatial Data: It includes Vector Data (points, lines, polygons) and Raster Data (grid-based data like temperature and elevation).
- Applications: Used across industries such as urban planning, agriculture, disaster management, and healthcare for decision-making and optimization.
Data Collection Methods: Includes GPS, remote sensing, surveying, crowdsourcing, and sensor networks. - Challenges: Issues include data accuracy, integration, privacy concerns, and resource limitations.
What Is Geospatial Data?
Geospatial data refers to information that is linked to specific locations on Earth. This data is primarily represented through coordinates such as latitude and longitude but can also be derived from maps, satellite images, and GPS systems. Geospatial data allows us to identify, analyze, and visualize the relationship between different objects, regions, or phenomena on the Earth’s surface.
Different Types of Geospatial Data
Geospatial data can be classified into two main types:
- Vector Data:
- This type of data represents geographic features as points, lines, and polygons. For example, a road network (lines), a city (point), or a park (polygon).
- Applications: Used for mapping and analysis tasks that involve boundaries or routes.
- This type of data represents geographic features as points, lines, and polygons. For example, a road network (lines), a city (point), or a park (polygon).
- Raster Data:
- Raster data consists of pixels or cells that form a grid, each representing a value. These are often used to represent continuous data like temperature, elevation, or land cover.
- Applications: Common in environmental monitoring, satellite imaging, and remote sensing.
- Raster data consists of pixels or cells that form a grid, each representing a value. These are often used to represent continuous data like temperature, elevation, or land cover.
Importance of Geospatial Data in Decision-Making and AI
Geospatial data is crucial for informed decision-making, particularly in industries like urban planning, logistics, agriculture, and disaster management. In geospatial data analysis, this data is used to train machine learning models for tasks such as predictive analysis, route optimization, and image recognition.
Methods of Geospatial Data Collection
There are various methods used to collect geospatial data, each suited for different purposes and technologies. These methods are essential in ensuring the accuracy and completeness of the data gathered. Understanding the benefits of data collection can help organizations choose the most effective techniques for their specific needs.
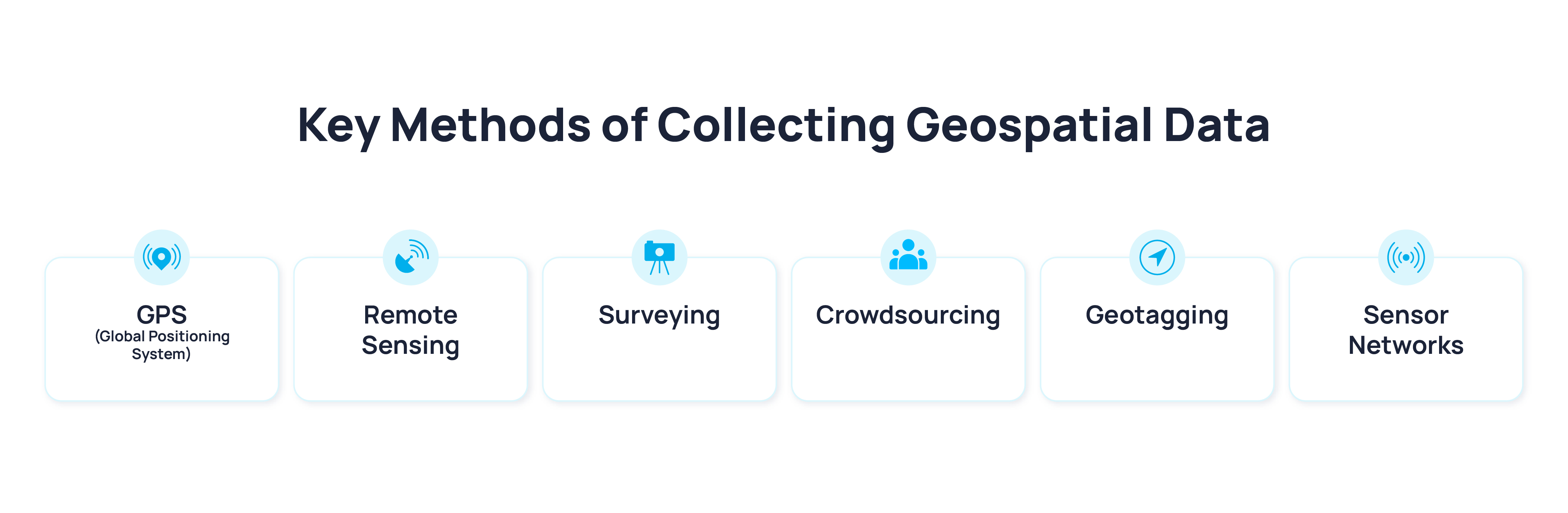
- GPS (Global Positioning System):
- GPS is widely used for determining the location of a point on the Earth’s surface using satellite signals.
- Real-World Application: Navigation apps like Google Maps, location-based services in smartphones.
- Remote Sensing:
- Involves collecting data from a distance using satellite or aerial imagery to observe and measure the Earth's surface.
- Real-World Application: Weather forecasting, land use monitoring, and disaster response.
- Surveying:
- Surveying uses instruments like total stations, theodolites, and levels to gather precise measurements of physical features.
- Real-World Application: Land development, construction planning, and infrastructure management.
- Surveying uses instruments like total stations, theodolites, and levels to gather precise measurements of physical features.
- Crowdsourcing:
- This method collects data from a large number of participants, often via smartphones or online platforms.
Real-World Application: Collecting real-time traffic data, citizen mapping projects.
- This method collects data from a large number of participants, often via smartphones or online platforms.
- Geotagging:
- The process of embedding location data into digital content such as images, videos, or social media posts.
- Real-World Application: Social media platforms like Instagram, geographic tagging in photos.
- Sensor Networks:
- Networks of distributed sensors collect data about environmental conditions (e.g., temperature, humidity, air quality).
- Real-World Application: Smart cities, IoT applications in agriculture, and climate monitoring.
Technologies Involved
- Satellites: Used for remote sensing to capture large-scale environmental data.
- Drones: Provide high-resolution images and data for small-scale or high-risk areas.
- IoT Devices: These sensors are integral in collecting data in real-time for smart cities and autonomous systems.
Applications of Geospatial Data
The versatility of geospatial data extends across a multitude of industries, driving efficiency, innovation, and smarter decision-making. The application of geospatial data can be seen in several key sectors that leverage its ability to map, analyze, and optimize resources and operations.
Geospatial Data in Analysis
Software tools have revolutionized geospatial data analysis, allowing users to transform raw data into meaningful insights. Similar tools are integral for professionals working with geospatial data in fields such as urban planning, environmental science, and healthcare.
Spatial Data Modeling and Big Data Integration
- Spatial Data Modeling: This involves creating models that represent real-world geographical phenomena.
- Big Data Integration: Combining geospatial data with other big data sources allows for deeper insights and more accurate predictions.
- Example: Combining climate data with geospatial data to predict areas at risk of wildfires.
Importance of Visualization Tools
- Mapping and Visualization: Tools like ArcGIS and QGIS enable users to create intuitive visual representations of geospatial data, making complex datasets easier to understand and analyze.
- Example: Heat maps to visualize areas with the highest traffic congestion or pollution levels.
Challenges in Working with Geospatial Data
While geospatial data offers significant benefits, working with it comes with several challenges that need to be addressed.
- Data Accuracy and Resolution:
- High-resolution data can be expensive and challenging to acquire.
- Solution: Leveraging crowdsourced data and sensor networks can help supplement traditional methods.
- Data Integration:
- Combining data from different sources (e.g., remote sensing, IoT) can be difficult due to varying formats and scales.
- Solution: Advanced data integration platforms can standardize geospatial data and facilitate analysis.
- Privacy Concerns:
- With geospatial data often tracking individuals’ locations, privacy becomes a major concern.
- Solution: Implementing secure data protocols and adhering to privacy laws ensures the responsible use of geospatial data.
- Resource Limitations:
- Gathering, processing, and storing geospatial data can require significant resources.
- Solution: Cloud-based platforms and decentralized data collection networks can help manage large-scale data requirements efficiently.
Why Choose Sapien for Your Geospatial Data Needs
At Sapien, we understand the complexities of geospatial data collection and analysis. Our scalable, decentralized workforce is capable of handling vast datasets with precision and accuracy. We provide high-quality data collection services across multiple domains, ensuring that your AI models are powered by the most reliable and accurate geospatial data.
Know more about Sapien’s Geospatial Data Collection Services
Key Benefits of Working with Sapien
- Scalable Workforce: Sapien’s network of over 80,000 contributors ensures that we can meet any project’s scale quickly and efficiently.
- Gamified Platform: Our unique gamified approach incentivizes high engagement and accuracy from contributors.
- Customized Data Solutions: We offer tailored data collection services that meet the specific needs of your project.
- Quality Assurance: Our multi-layered quality assurance process ensures that every piece of data meets the highest standards.
Partner with Sapien, a leading data integration platform, to unlock the full potential of your geospatial data and drive better business insights.
FAQ
What are some examples of geospatial data?
Geospatial data examples include satellite images, GPS coordinates, land use maps, elevation data, and weather patterns. These can be collected through methods such as remote sensing, crowdsourcing, and sensor networks.
What is the difference between geospatial data and spatial data?
Geospatial data refers to data linked to specific geographic locations, including information like latitude and longitude. Spatial data, on the other hand, is a broader term that refers to any data that describes the position, size, shape, or orientation of objects in space, including both geographic and non-geographic data.
What challenges do organizations face when integrating geospatial data into their existing systems?
Organizations face challenges such as data compatibility issues, the need for high-resolution data, difficulty in integrating multiple data sources, privacy concerns, and the need for specialized software and skills to process and analyze the data efficiently.